Happy Thursday and welcome to Patent Drop!
Today, a patent from Microsoft could prevent people from trying to “jailbreak” large language models. Plus: a JPMorgan Chase patent takes on bias in lending, and IBM wants to find out what’s got you under the weather.
Before we jump into today’s patents, a word from our sponsor, Brex. Any successful startup founder knows that runway is everything. Stretching each dollar is crucial, which is why 1 in 3 US startups use Brex. With Brex you get the best of checking, treasury, and FDIC protection in one account — learn more about Brex’s banking solutions.
Let’s jump in.
Microsoft’s Chatbot Filter
If you don’t have anything nice to say, don’t say anything at all. At least not to chatbots.
Microsoft filed a patent application for “adverse or malicious input mitigation for large language models.” The company’s filing details a system to prevent users from feeding a language model inputs that would elicit outputs that are inappropriate or present security risks.
The tech essentially aims to keep models from hallucinating and spitting out inaccurate answers, as well as avoid prompt injection attacks “in which user inputs contain attempts by users to cause the LLMs to output adverse (e.g., malicious, adversarial, off-topic, or other unwanted) results or attempts by users to ‘jailbreak’ LLMs.”
When a language model receives a malicious input, the system will pull similar pairs of bad inputs and their corresponding appropriate responses from a database of past interactions. Additionally, it may pick inputs that are similar to the conversation, but not malicious, so the model learns the difference between normal and inappropriate inputs.
Those similar pairs are incorporated into the language model’s input, essentially filtering user chats before they reach the language model. That way, the model has an example of how to correctly respond to a malicious input, and it’s dissuaded from answering in an unsafe way.
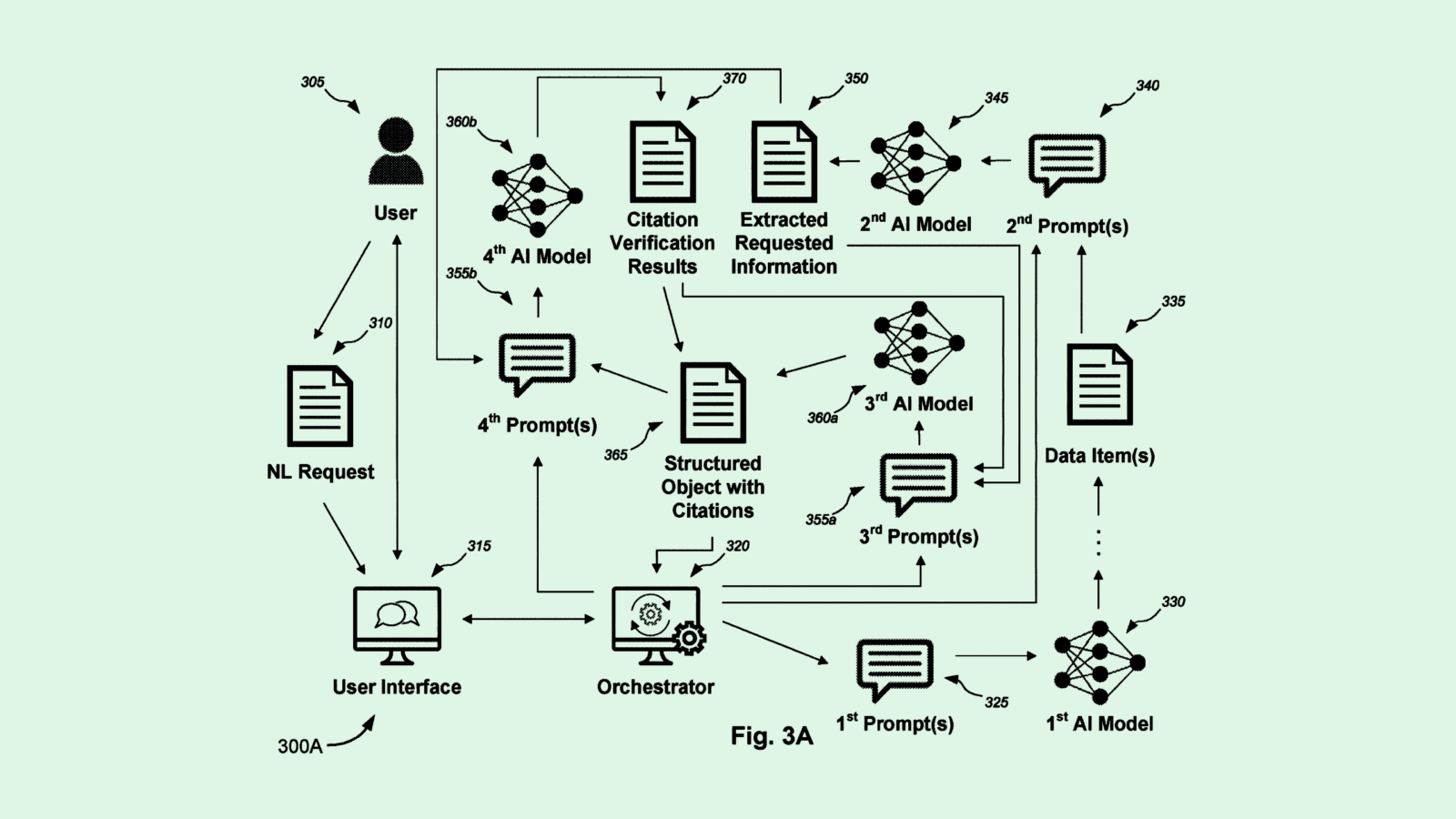
It makes sense why Microsoft would want to keep its models from spilling the beans. The company has spent the past year promoting its AI-powered work companion, Copilot, as a means of boosting productivity. And though it has a partnership with OpenAI, Microsoft is also developing its own language models, both large and small. As the AI race continues to tighten, any security breakdowns could be massively detrimental.
Though there are a lot of ways to protect AI models, monitoring user behavior is a vital piece of the puzzle, said Arti Raman, founder and CEO of AI data security company Portal26. Most malicious activity related to AI models comes from prompt-based attacks, she said. Because of this, these attacks are a major talking point among model developers and tech firms.
“There are different types of ways in which [large language models] can be attacked, and malicious users are a pretty important one,” said Raman. The problem is multiplied if a user’s prompts are used to further train a model, as the attack “lives on in perpetuity,” she said. “It becomes part of the model’s knowledge, and just creates an increasing and exploding problem.”
There are three primary ways to keep an AI model from leaking private sata, said Raman. First is by protecting the data pipeline itself, in which data security faults are intercepted before they reach the model. The second, demonstrated in Microsoft’s patent, is using pattern recognition and user monitoring to mitigate attacks in real time. The third is identifying commonly-used malicious prompts and robustly protecting against them.
The best approach, especially as AI development and deployment reaches a fever pitch, is a combination of the three, she said. “We should throw everything we have at the problem so we make sure that our pipelines are protected up front,” Raman said.
Extend Your Runway, Scale Your Business
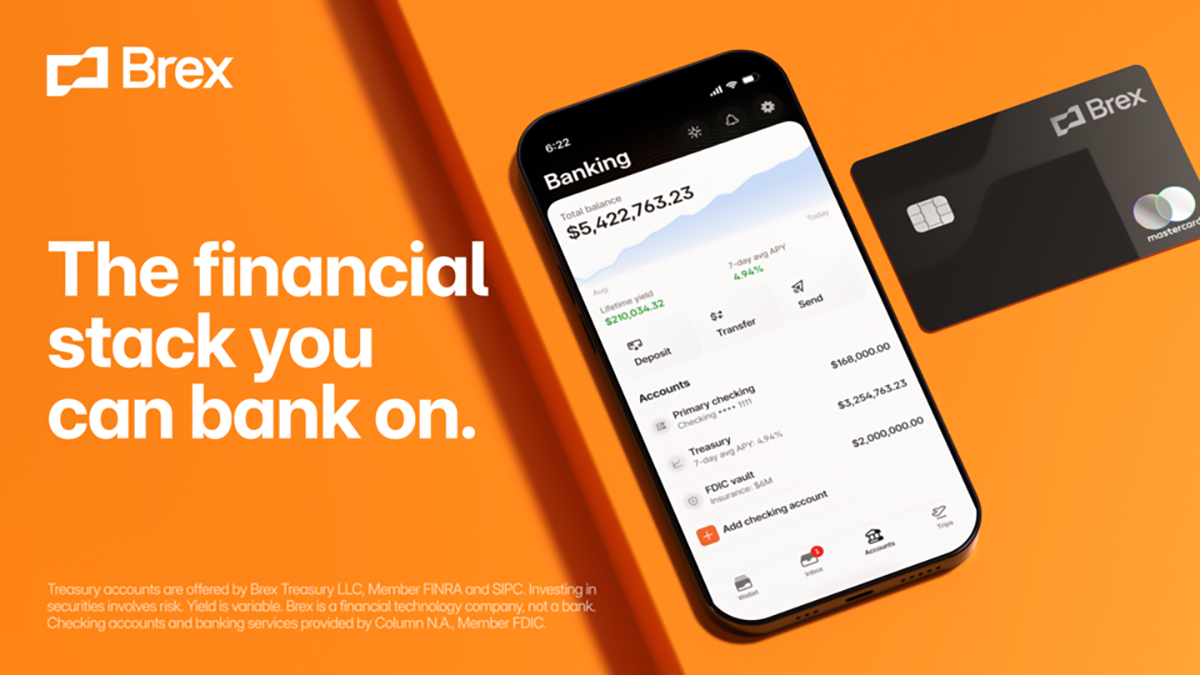
A long runway is crucial for two types of people: pilots and startup founders.
Fortunately, airports tend to have well-maintained runways, but it’s a different story for founders. Why do most banks chip away at their funding with fees, minimums, and delays? Founders deserve better — that’s why Brex built a banking solution that helps startups take every dollar further.
With Brex, you get the best of checking, treasury, and FDIC protection in one account:
- Send and receive money fast worldwide.
- Earn industry-leading yield from your first dollar — while being able to access your funds anytime.
- Protect your cash with up to $6M in FDIC coverage through program banks.
Ready to join the 1 in 3 US startups using Brex and take every dollar further?
JPMorgan Chase Catches Lending Bias
JPMorgan Chase may want to track when its algorithms are making questionable decisions.
The financial institution filed a patent application for “algorithmic bias evaluation of risk assessment models.” JPMorgan’s tech tracks when risk assessment models — used to determine the risks associated with lending and investment — are acting unethically or exhibiting bias.
“There has been a growing focus on model bias, including fairness in the provision of financial products and services beyond credit and, although the focus on model bias has been increasing in recent years, this trend has accelerated over the past year,” the bank said in its filing.
First, a model is evaluated to get a sense of its predictions and assessments. The results are transmitted to a “disparate impact analysis service,” which is responsible for analyzing the model’s score for ethical standards and bias.
Then, the analysis service connects with a “government monitoring information” database, which picks out information related to demographics such as ethnicity or gender. That data corresponds with what the filing calls “ethics and compliance initiative” information, which tracks how a model stacks up to ethical or regulatory standards.
Finally, the analysis service analyzes the model’s score compared to the demographic information and ethical standards to determine whether the risk assessment model is operating as it should. JPMorgan noted that this service is scalable for models of all sizes.
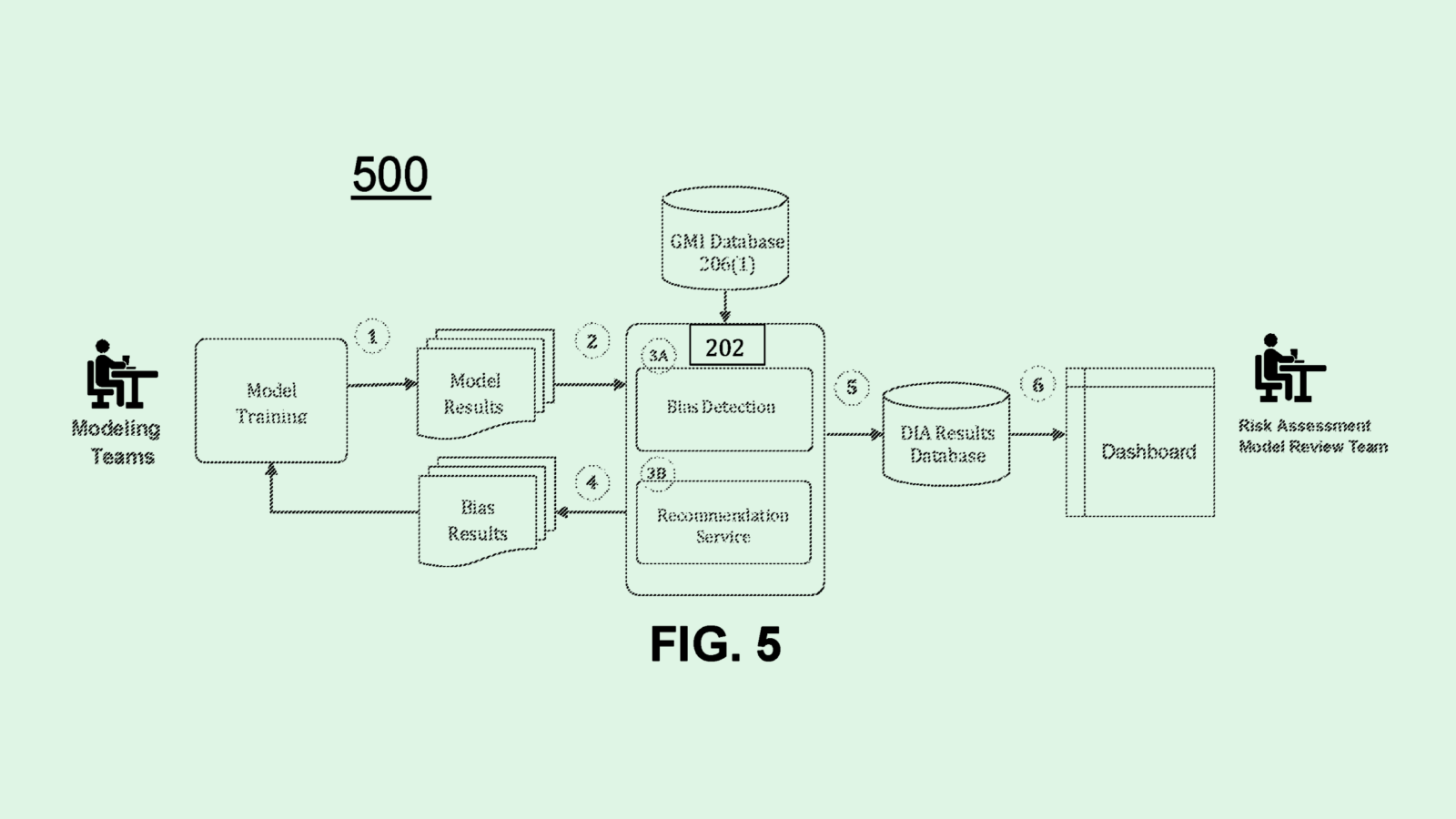
JPMorgan has been loud about its keen interest in AI. The company’s patent history is loaded with IP grabs for AI inventions, including volatility prediction, personal finance planning, and no-code machine learning tools. The company also launched an AI-powered cash-flow management tool, a product called “IndexGPT” for thematic investing, and rolled out an in-house AI assistant called LLM Suite to its more than 60,000 employees.
But a growing footprint in AI increases risk — especially when it comes to lending and risk analysis. Bias has long been an issue in AI-based lending tools, which often discriminate against marginalized groups. In some contexts, the issue has caught the attention of regulators: The Consumer Financial Protection Bureau in June approved a rule that ensures accuracy and accountability of AI involved in mortgage lending and home appraisal.
By not tracking bias in AI models, especially customer-facing ones, financial institutions create “economic headwinds” for specific groups, said Brian Green, director of technology ethics at the Markkula Center for Applied Ethics at Santa Clara University. This opens them up to risks of both regulatory clapback and reputational harm.
“Bias means that your machine learning model is messed up — it’s not working correctly,” said Green. “Ultimately, it ends up creating bad business. There are lots of places where automated systems do bad things to people, and it’s very hard to get those things fixed.”
Patents like these may hint that JPMorgan is seeking to mitigate bias before models reach customers’ hands — though locking down IP on inventions aimed at fighting bias may prevent other financial institutions from bettering their own systems in this way, Green added.
IBM’s Medical Degree
IBM may want to help doctors make quicker diagnoses.
The tech firm filed a patent application for a way to use a generative adversarial network, or GAN, for “electronic health record extrapolation.” IBM’s filing outlines a way to train and utilize an AI-based patient diagnostic tool.
Current diagnostic models come with a number of problems, IBM said. Some are tedious and expensive to train, yet are “limited by disease rarity” due to limited training data, the filing notes. Others may have a wider net of training data, but yield results that are “often trivial and not-disease specific.”
To create a more robust diagnostic tool, IBM’s tech relies on a generative adversarial network, which uses two models — one to generate artificial data and another to discriminate between real and fake until the two are indistinguishable — to improve predictions. This network is trained on the authentic health records of real patients.
Once the network is trained, it can be applied to new patient health records, and predict both possible diagnoses of current conditions as well as “latent diagnoses,” which may appear later, based on predicted disease trajectory.
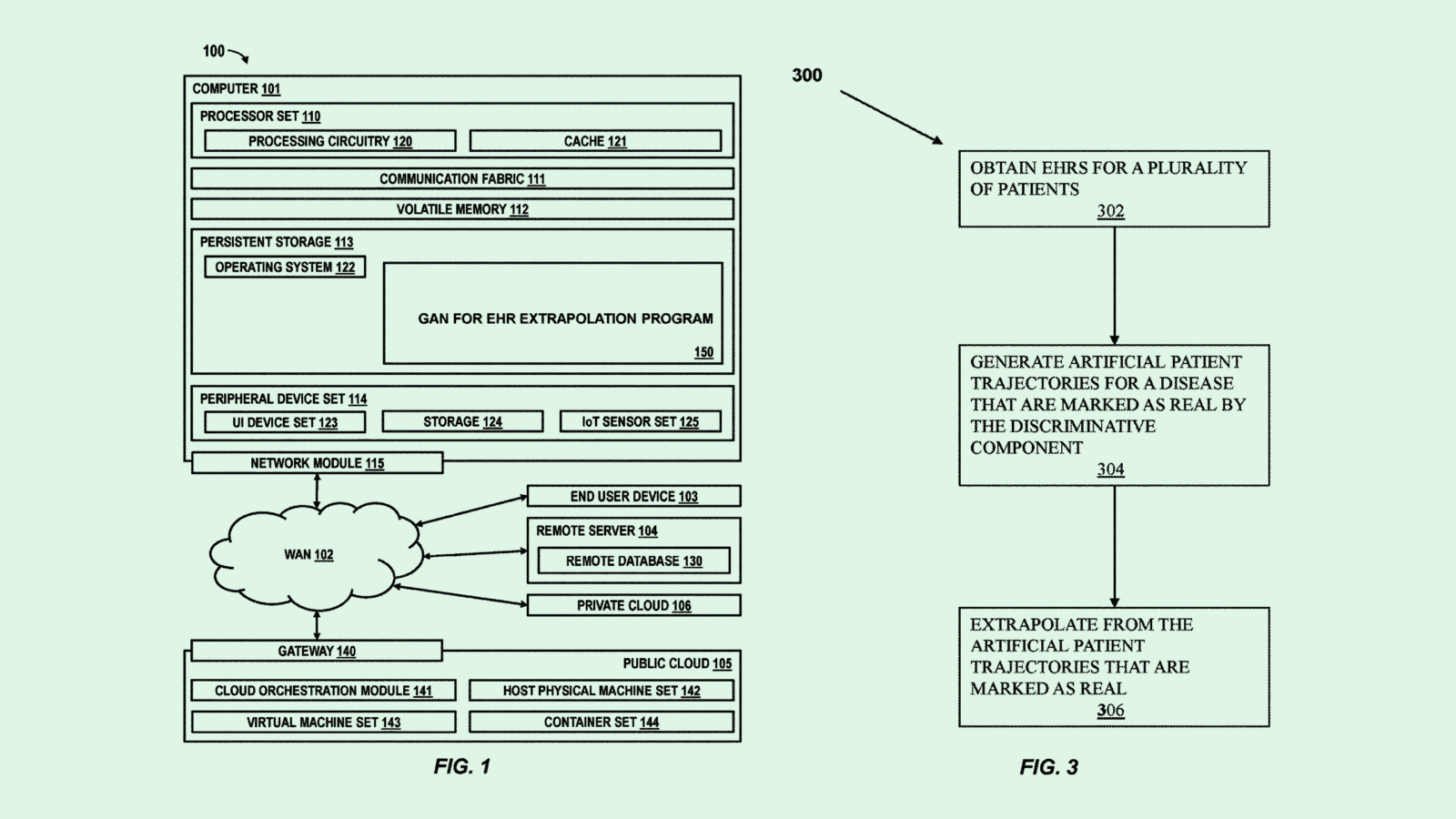
IBM isn’t the first to explore machine learning’s place in healthcare. Medical device firm Philips has sought to patent AI-based systems that predict emergency room delays and reconstruct medical imagery. Google filed a patent application for a way to break down and analyze doctors’ notes using machine learning. On the consumer end, Apple has researched ways to put the Vision Pro to use in clinical settings using a “health sensing” band.
AI has a lot of promise in the healthcare industry in areas such as documentation, imaging, and quicker diagnostics as staffing shortages loom. The problem, however, is the data. For one, in the US, healthcare data is strictly protected under the Health Insurance Portability and Accountability Act (HIPAA).
And given that AI needs a lot of high-quality data to operate properly, it’s particularly difficult to build models for healthcare settings. Though synthetic data may present a solution, because these models are being used in an area where accuracy is important, authentic data would likely allow for a more robust model — especially since lacking data leads to the dreaded issue of hallucination.
The other problem lies in how data is treated beyond training. Because AI has known data security problems, it may be difficult to get patients to trust models with their most sensitive information.
Extra Drops
- Roku wants to know if you’re in need of help. The company filed a patent application for the collection and reporting of “customer premises context information” in response to a predicted emergency.
- Snap wants you to smile. The company filed a patent application for “photorealistic real-time portrait animation.”
- Sony wants to keep your game from glitching. The company filed a patent application for methods to “reduce latency in cloud gaming.”
What Else is New?
- Microsoft is cutting 650 employees in its Xbox gaming division, with the cuts impacting “mostly corporate and supporting functions.”
- British Technology Minister Peter Kyle labeled data centers critical infrastructure in a move that’s aimed at boosting cybersecurity.
- Brex Knows That Runway is Crucial. So they built a banking solution that helps founders take every dollar further. Unlike most traditional banking solutions, Brex has no minimums and gives you access to 20x the standard FDIC protection via program banks. And you can earn industry-leading yield from your first dollar — while being able to access your funds anytime. Get Brex, the business account used by 1 in 3 US startups – learn more.*
* Partner
Patent Drop is written by Nat Rubio-Licht. You can find them on Twitter @natrubio__.
Patent Drop is a publication of The Daily Upside. For any questions or comments, feel free to contact us at patentdrop@thedailyupside.com.