Happy Monday and welcome to Patent Drop!
Today, a cloud privacy patent from Amazon highlights the growing movement toward data repatriation, and the tricky situation it presents for cloud providers. Plus: Meta makes AI more private and personal; Salesforce ups its language skills.
Let’s check it out.
Amazon Keeps It Hidden
Amazon wants to keep private data private.
The company filed a patent application for “isolation techniques at execution platforms used for sensitive data analysis.” Amazon’s tech aims to silo private data used in application development, such as building AI, in order to protect it while still allowing developers to build with it and third-party applications to use it.
“Many applications may involve analysis and processing of sensitive data sets of the clients, and preventing the misuse or misappropriation of such data may represent a non-trivial requirement,” Amazon said in the filing. This also applies to “protecting … application code (e.g., from being stolen or copied.)”
To address this, Amazon’s tech separates data into two different environments: The first handles all of the computing tasks themselves, and the second handles data flow and input.
The second environment acts as a “secure intermediary” for the data that goes between it and the first environment, as it is configured to meet “client-specified isolation requirements” for how sensitive data is accessed and used, and can only be accessed by specific users. It’s like a locked supply room, for which only a few people have a key.
Additionally, the first environment “may be ‘zeroed out’ or scrubbed after the application’s work is completed” for an additional layer of security.
This separation allows third-party developers to leverage the resources of a large cloud computing network, such as Amazon Web Services, without having to worry that their data, such as customer or personal information, is at risk or being breached or stolen.
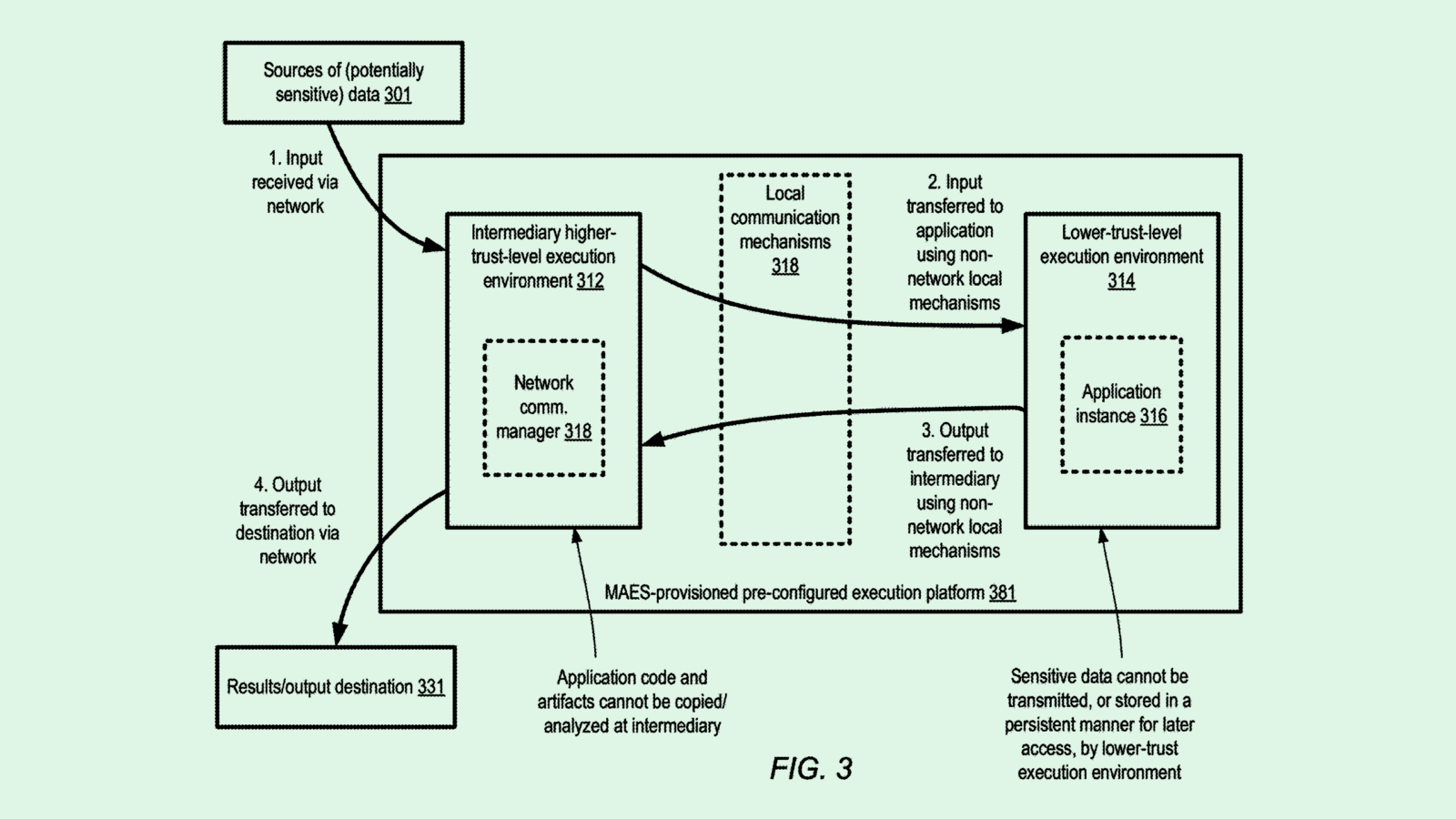
With the growing pace of AI adoption, data security is more important than ever, especially to larger enterprises, said Trevor Morgan, senior vice president of operations at OpenDrives. Security measures are a primary concern and “real differentiator” when picking a cloud environment for those that have to worry more about regulatory scrutiny and compliance, he said.
But across the board, many are questioning whether or not they need to rely on cloud services as much as they do — or if they even need them at all, said Morgan. It’s a movement called “data repatriation,” he said, with the goal of keeping as much of your own data in your own hands as possible.
“It speaks to this movement of people questioning, ‘Do I really want my most private IP sitting out there?’” said Morgan.
Of course, people looking to claw their data back could make AWS nervous, said Morgan, leading to a decreased reliance on cloud services. This patent may be a response to that growing movement, he said: It seemingly aims to showcase that tenants of cloud environments like the ever-popular AWS can trust that their data and intellectual property won’t be misappropriated.
“I think it is their insurance policy against the growing movement of data repatriation,” said Morgan. “To me, this just seemed like [Amazon] getting out in front of it.”
And even those that aren’t looking to pull out of cloud environments entirely are still trying to figure out a balance or the “perfect hybrid,” he said. Striking that balance is different for every enterprise. “There’s a time and place for both, and even the large companies are trying to figure out what that is,” Morgan said.
Meta Goes Private
As Meta attempts to keep up in AI, the company may want to get personal.
The social media giant filed a patent application for “group personalized federated learning.” Federated learning allows a global model to train locally on data from multiple devices without actually transferring that data from those devices.
However, the problem with this technique is that “typically, a majority of users only have a few training examples, making it challenging to improve the performance of a machine learning model for individual client devices,” Meta said in the filing.
To overcome this, rather than simply learning from each user, Meta’s tech uses federated learning techniques to group users’ models by their similarities.
When a global model is trained on local data from users’ devices, the trained model is sent back to a central server. The server analyzes the new models’ parameters to discover similar patterns to batch them together. Those batches are then used to create and deploy group-specific machine learning models customized to suit the characteristics — such as demographics or social media profile types — of each group. This allows Meta to train a model to be more robust without invading users’ privacy.
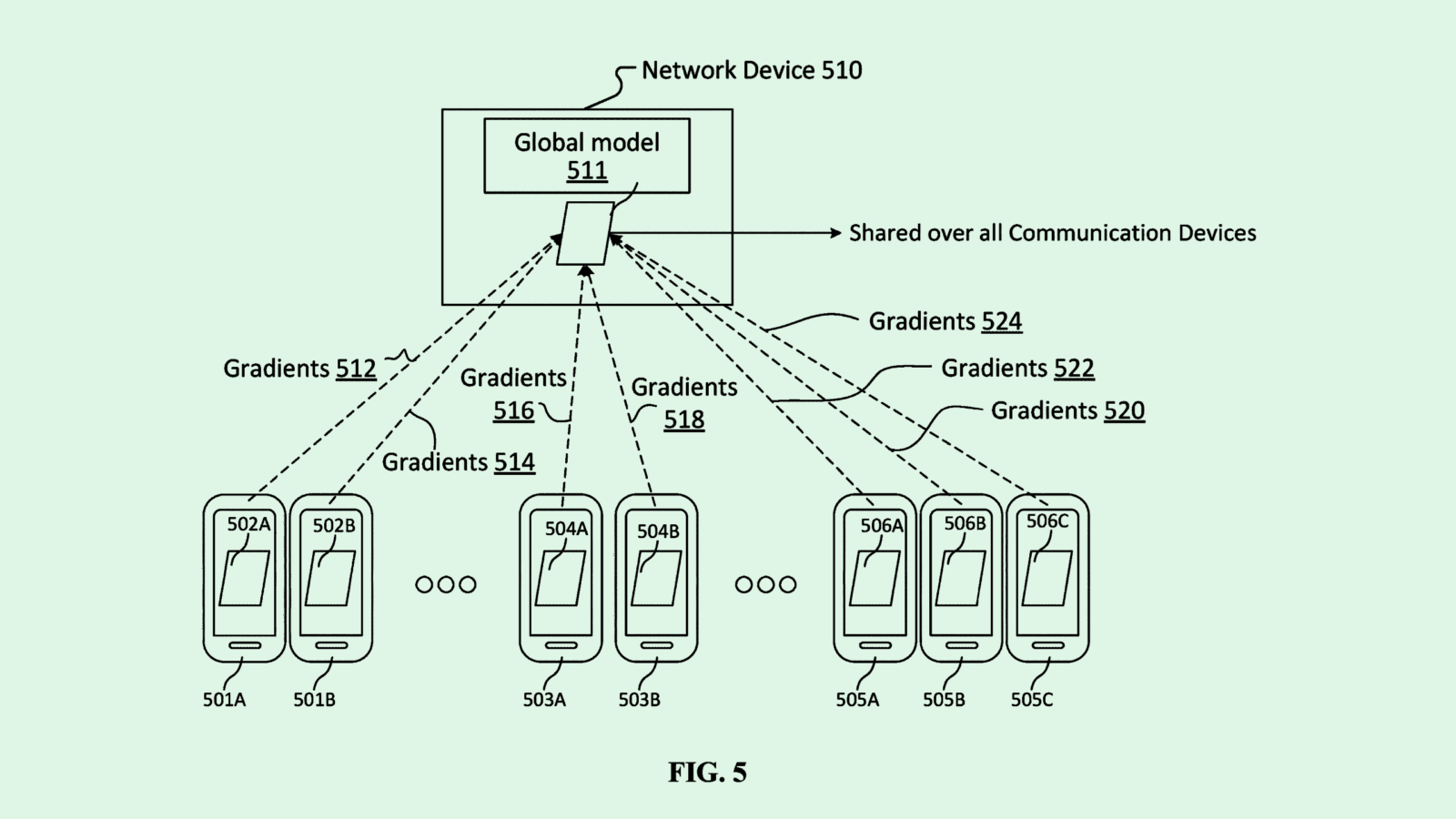
Federated learning is one of the most common forms of AI training, said Pakshi Rajan, VP of products and co-founder at AI data security company Portal26. While it’s helpful for training a global, general model without violating data privacy, Meta’s patent may present an improvement on this concept by allowing for localization and personalization, he said.
For example, if a self-driving car company is using federated learning to train its vehicles, this could help vehicles understand and apply local traffic laws. “My gut feel is that a lot of people are already doing this,” Rajan said.
In Meta’s case, there are several ways this can be useful. For starters, given that the company’s main cash cow is digital advertising, it could allow for personalized advertising based on profile demographics without compromising privacy. It could also help Meta personalize its AR experiences, Rajan noted, without necessarily exporting data from user headsets.
“Personalization can be done in such a way that the data can be sent without compromising the personal space of the person or the owner of the device,” said Rajan.
Meta, however, doesn’t necessarily have the best reputation for user privacy. And the company has acknowledged using all public user posts since 2007 (unless you’re in the EU) to train its models. Tech like this could keep its models from prompt attack data security slips — or at least give it some “thought leadership brownie points,” said Rajan.
Salesforce’s Duolingo
Salesforce is taking language classes more seriously.
The tech firm is seeking to patent a system for “cross-lingual transfer learning” of AI models. Salesforce’s tech aims to help language models complete tasks in less-common languages with the same accuracy as they would in more widespread languages like English, Spanish, or Chinese.
“Unfortunately, most [natural language processing] models are trained to perform conversation tasks using datasets in rich-sourced languages, e.g., English or a few other high-resource languages,” Salesforce said in the filing. “The ability to translate or communicate in less-sourced languages remains limited.”
Even if an underlying model is multilingual, it’s often difficult for these models to generalize well in other languages, Salesforce noted. To overcome this, this filing proposes a system to tune the prompts going into an AI model rather than its parameters.
Two prompts that mean the same thing in different languages are paired together and then “randomly masked,” meaning certain words and phrases are hidden, to teach the model to reconstruct them. In addition, a “soft prompt” is added to the beginning of both prompts before they are fed to the language model: That entails additional vectors, not necessarily interpretable by humans, that help the model understand the task.
The system measures how well the model does at piecing together the original prompts for each language, and uses that metric to retune the soft prompt. As a result, the model can effectively understand queries no matter their language.
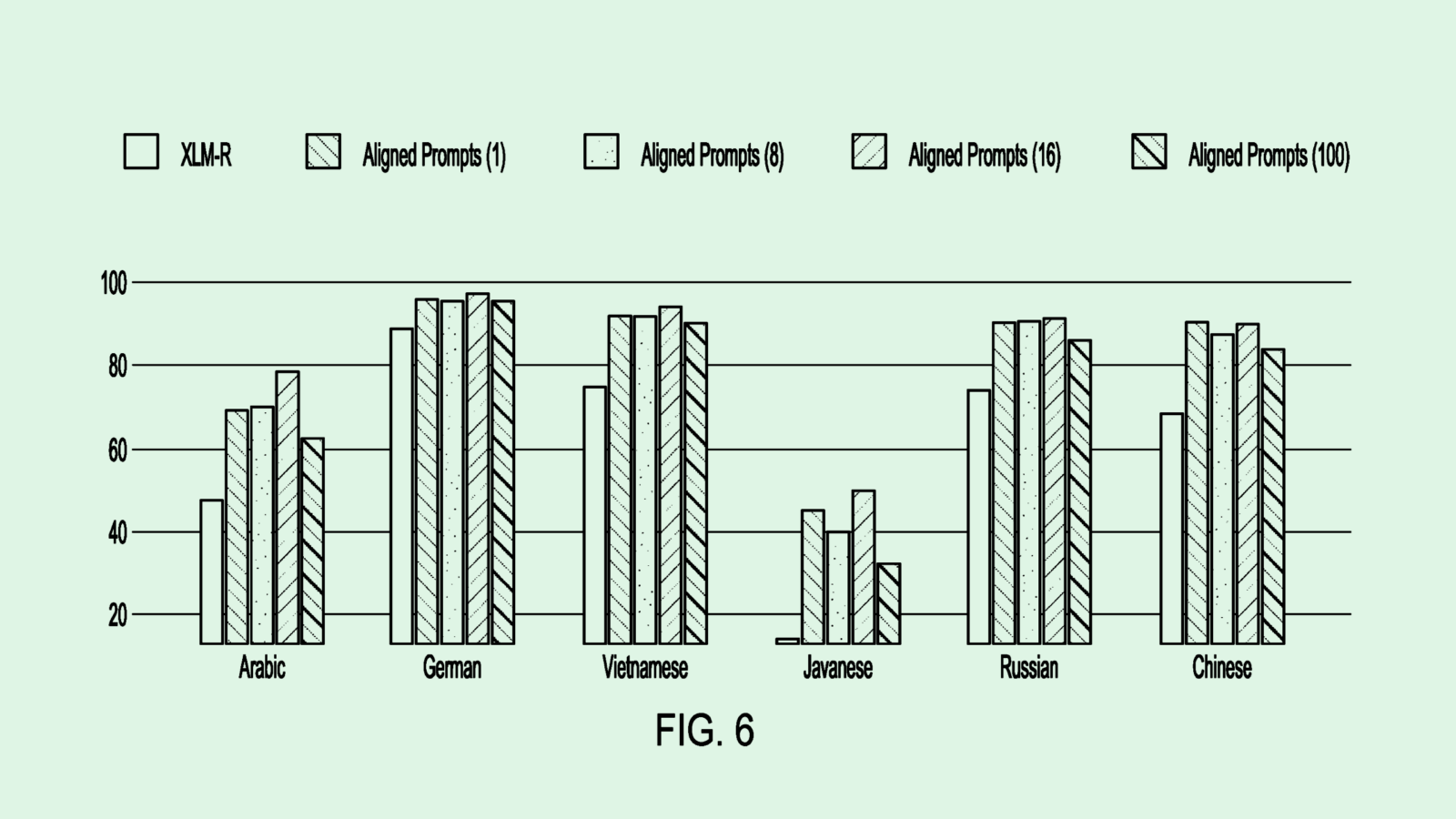
As it tries to garner the AI spotlight that the likes of Google, Microsoft, and OpenAI are hogging, Salesforce has placed a lot of emphasis on one particular implementation: AI agents. At Dreamforce last month, the company announced a new service called Agentforce, a customizable AI agent platform designed for customer service, sales, and marketing.
At the conference, CEO Marc Benioff called easy-to-use, personalized AI agents — without the hassle of customers needing to customize them — the “third wave of AI.”
Its patent history tells a similar story, which includes a filing for an easily customizable AI platform that learns from an organization’s internal data. And patents that strengthen AI’s language skills also make sense in the context of expanding AI agents: AI that only performs well in “high-resource languages” isn’t going to be useful for many people.
But Salesforce’s agent quest has caught the eye of competitors. Microsoft is launching its own set of 10 autonomous agent tools capable of handling emails, managing records, and other tasks for sales, customer support, and accounting roles. Anthropic also released its own AI agent this week in public beta, capable of “computer use.”
Extra Drops
- Intel wants to make sure it’s you behind the wheel. The company filed a patent application for “multi-modal context based vehicle theft detection.”
- Roblox wants to make sure everyone’s playing fair. The company wants to patent a system for “in-environment reporting of abuse.”
- Snap is becoming an optometrist. The social media firm filed a patent application for “eyewear with inter-pupillary distance estimation.”
What Else is New?
- Apple released a beta version of Apple Intelligence on Wednesday, including an integration with ChatGPT.
- Tesla’s Cybertruck was the third best-selling EV in the U.S. in the third quarter, surpassing every other non-Tesla EV.
- Roblox will roll out child-safety rules on its platform, allowing parents to monitor their children’s online activity.
Patent Drop is written by Nat Rubio-Licht. You can find them on Twitter @natrubio__.
Patent Drop is a publication of The Daily Upside. For any questions or comments, feel free to contact us at patentdrop@thedailyupside.com.