Happy Monday and welcome to Patent Drop!
Today, Google’s recent patent to boost AI efficiency signals that massive models may cost more resources than they’re worth. Plus: Stripe wants to make crypto payments seamless as it steps back into the market, and Alibaba wants to shave down its neural networks.
Ahead of today’s Patent Drop, we want to direct you to this free guide from Persona about Know Your Business (KYB). While the traditional KYB process isn’t ideal, it doesn’t have to be so manual and error-prone. Download Persona’s guide to learn how to build a more effective process today.
Now, let’s jump into it.
Google’s AI Trade-off
Google may be looking for ways to cut down its AI power bill.
The company filed a patent application for “contrastive pre-training for language tasks.” The goal is to provide an alternative to “masked language modeling” approaches that typically suck up a lot of energy when training language models.
“An important consideration for pre-training methods should be compute efficiency rather than absolute downstream accuracy,” Google said in the patent.
When using masked language modeling for training, models guess a missing (or “masked”) word based on the context of the surrounding ones. These approaches typically mask a small subset of words — around 15% — in a set of data to teach the model to recover those missing words.
Conversely, Google’s method of contrastive training helps language models differentiate inputs from other plausible alternatives. Similar to masked language modeling, at the start of the process, a certain subset of words are masked prior to training. Then a “generator” model creates replacement words for the masked ones.
Finally, the main model is trained to predict whether the word in the sentence is real or AI-generated, helping it understand language better. This method is faster, more cost-effective, and more energy-efficient, as the objective is simpler than that of masked modeling, and the model only has a small subset of data to work on.
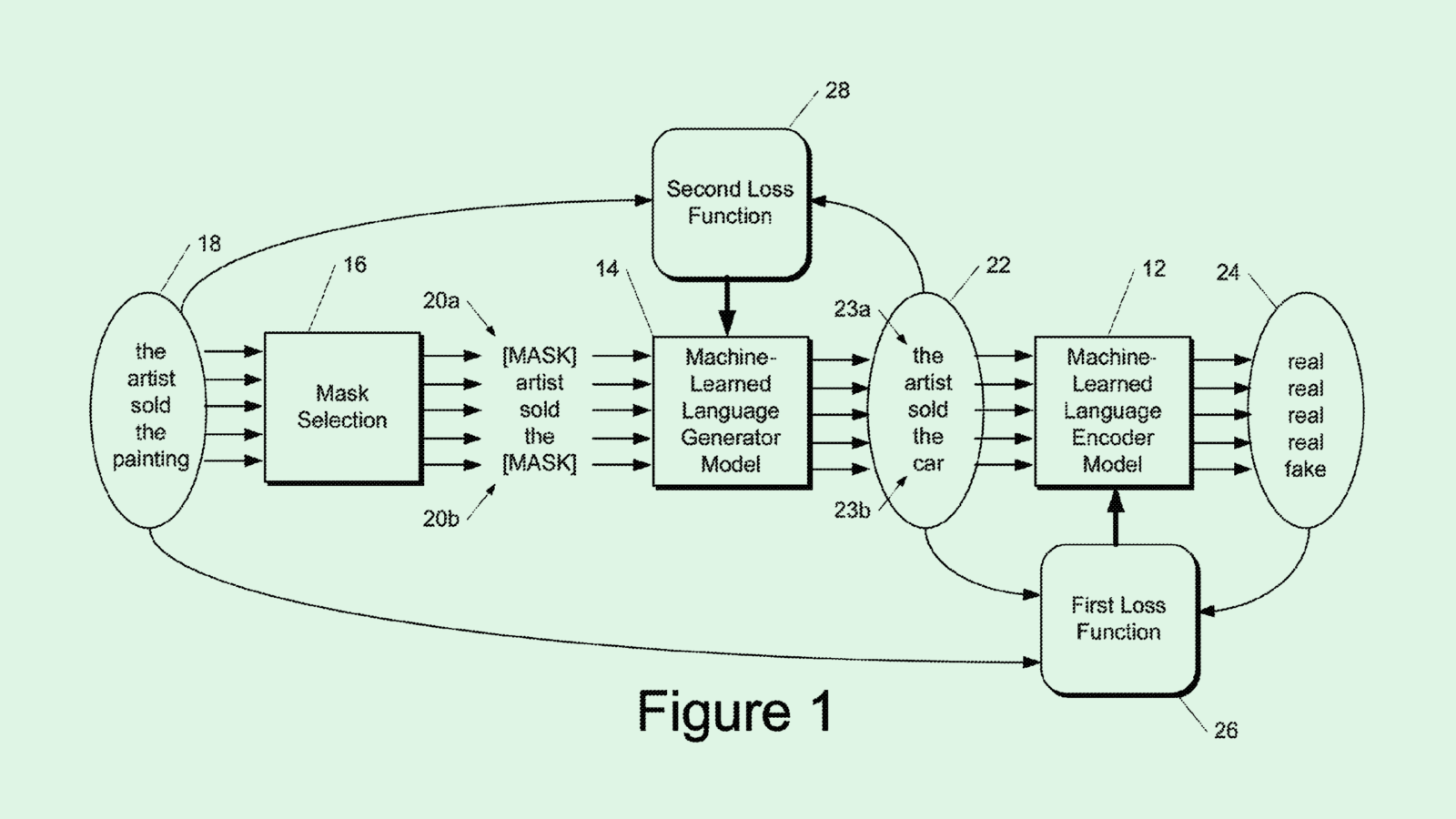
Google’s I/O event last week was packed with announcements, from AI search overviews to image analysis tools to AI personal assistants. Many of these tools were backed by Gemini, the company’s massive foundational AI model. But despite tech firms’ lofty promises about AI capabilities, models like Gemini are often simply too big for the average person or company to get consistent use out of them, said Vinod Iyengar, VP of product and go-to-market at ThirdAI.
Using a model like Gemini for every AI-related task is costly in both computing power and cash. Plus, many tasks don’t require the power that Gemini has, he noted. Infusing massive models into simple operations is like using an ax to cut a tomato.
So why would tech firms pour millions into these models in the first place? The simple answer: Because they can, Iyengar said. AI models with billions of parameters help companies like Google and OpenAI show that they have the “best in class” as a way to draw customers, he said.
However, those customers will likely end up using smaller, resource-efficient, and lower-latency models offered by these tech firms. “It’s a capability demonstrator,” said Iyengar. “But there is no real use case because people for the most part are going to use those smaller models.”
Patents like these show that the tide is shifting toward these models, said Iyengar. Despite the incredible capabilities of something like Gemini, the mounting cost of AI development will shift the paradigm from wanting the best to wanting “good enough” at the best price, he said.
“They’re kind of signaling to the market two things: We have the capability to build the most monstrous model that you can imagine, but we also have these great tier two models, which are very fast and very cheap,” said Iyengar.
The practical guide for building a highly effective KYB process
Historically, the Know Your Business (KYB) process has been extremely manual, disjointed, and complex. Think: manually collecting information, going back and forth between customers, and needing to piece together multiple point solutions.
That’s why Persona wrote the practical guide for building a highly effective KYB process — to empower leaders like you to streamline KYB, meet ever-changing regulations, fight fraud, and build trust.
In Persona’s free ebook you’ll:
- Learn the key components and considerations of an effective KYB process
- See what KYB looks like in action
- Read how leading fintechs and marketplaces use Persona to streamline KYB-KYC compliance
Download the ebook to start streamlining your process today.
Stripe Plays Crypto Catch-up
After a six-year break, Stripe is back in cryptocurrency. A recent patent shows that it may have been plotting its comeback for a while.
The company filed a patent application for a “fiat-crypto onramp.” This patent, filed originally in late 2022, aims to make crypto transactions faster and more seamless by using a “proxy cryptocurrency wallet” and pre-authorization techniques to ensure that a user has enough funds for their purchases.
Stripe noted that the friction and complexity of crypto transactions can be a turn-off for consumers. “This delay, confusion, and poor integration can often lead to user drop-off and abandonment of the purchase, thereby making it difficult for entities in the cryptocurrency ecosystem to make sales using cryptocurrency, especially when working with consumers who are new to cryptocurrency transactions,” the company said.
When a user initiates a crypto transaction, their crypto wallet first sends a pre-authorization to a proxy wallet, which includes details about the transaction such as what’s being purchased and the cost. Upon receiving the request, the proxy wallet checks whether or not the user has enough crypto to complete the transaction.
If they do, the transaction is executed. If the user doesn’t have sufficient funds, this system will automatically purchase the remaining balance for them using the bank information tied to their account. This streamlines the process of making purchases with crypto, as it cuts out the additional step of the user needing to ensure their wallet is properly stocked beforehand.
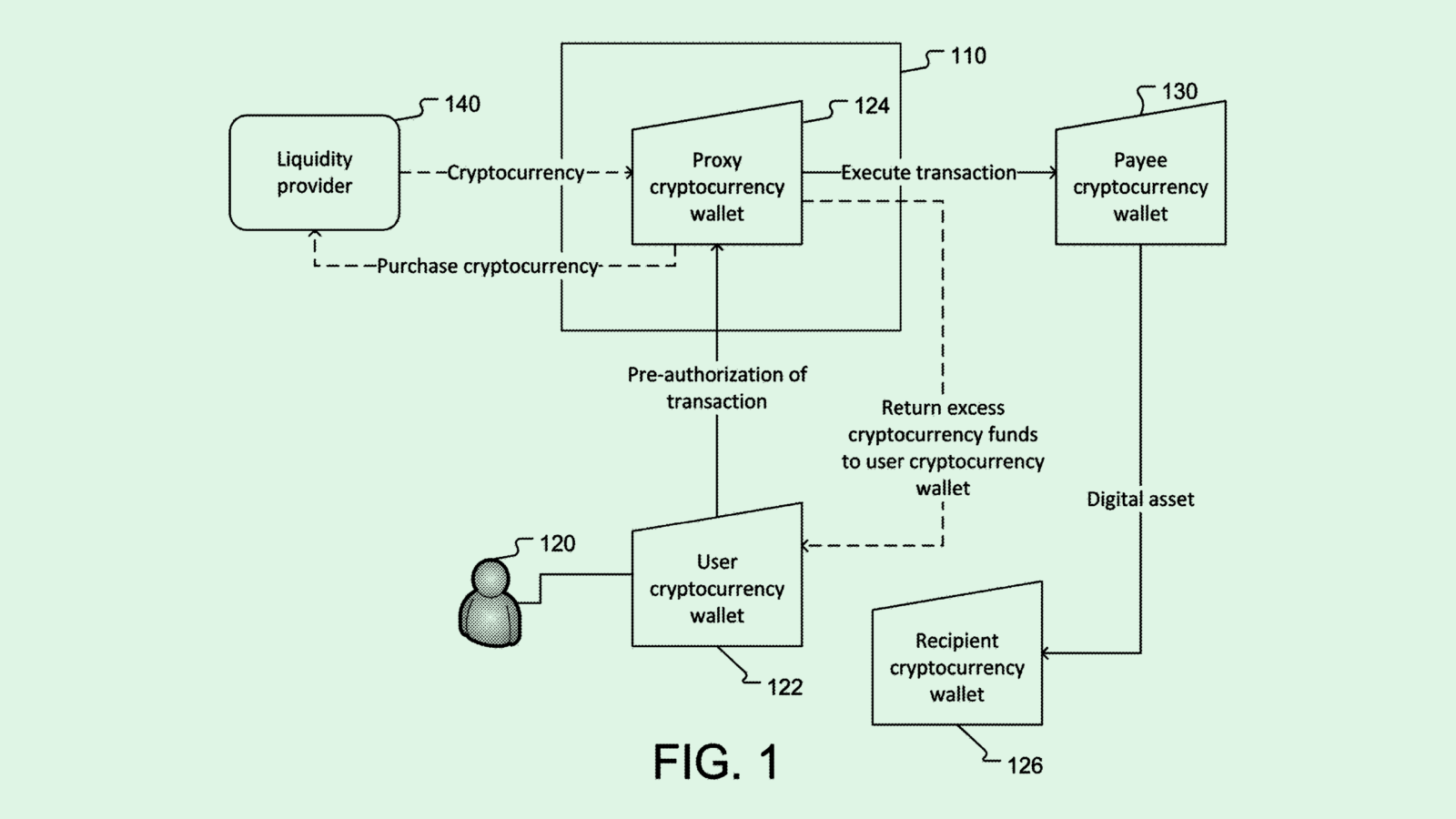
After putting its crypto plans on ice in 2018, Stripe will again allow customers to accept digital currency as payment, starting solely with USDC stablecoins, a digital currency pegged to the dollar.
“Transaction settlements are no longer comparable with Christopher Nolan films for length,” Stripe Co-founder and President John Collison said during the announcement last week. “And transaction costs are no longer comparable with Christopher Nolan films for budget.”
Stripe’s return could help restore some confidence in the crypto market after a tough few years, said Jordan Gutt, Web 3.0 Lead at The Glimpse Group. The company is one of the largest payments processors in the world, and its comeback could “play a role in increasing the standard and trust around cryptocurrency,” Gutt noted. “I think they’re preparing for a potential bull run for crypto.”
Starting with stablecoins is also a smart move, said Gutt. “Stablecoins are great because, well, they’re stable. They’re not volatile like Ethereum and Bitcoin and many others.”
Stripe may be aiming to make it as easy as possible for people to access and actually use digital currencies, Gutt said. The seamless exchange and on-ramp between fiat currency and crypto make it so the customer doesn’t have to go through extra steps to use their crypto, he said, and opens up the doors for merchants to get involved in blockchain more easily.
Plus, this would likely be integrated directly into Stripe’s existing systems, he said, making accepting and using crypto an easy add-on. “A huge part of it is the user experience, which is already great for Stripe,” said Gutt. “They’re able to widen the market for people who want to use crypto for payments, making it more trustworthy and being able to do it at scale.”
Alibaba’s Shrink Ray
Alibaba wants its AI models to be a touch smaller.
The company filed a patent application for a system for “reducing (the) size of a neural network model.” Alibaba’s system looks to reduce the data storage and computational requirements of neural networks, which tend to be much larger and compute-intensive than other models.
While conventional systems can shrink models using pruning strategies, or removing parameters, these systems often have “little consideration of how to trade off between model accuracy and size reduction with the same execution efficiency,” Alibaba said in the filing.
Alibaba’s model compression method involves determining the size of the available hardware’s “vector register,” or essentially understanding the capabilities and data storage capacity of the hardware the neural network will run on.
Then the system compresses the data in the model to fit neatly into this vector register by repeatedly pruning the model of excess data. This process is monitored after each round of pruning to understand how the model’s performance is impacted, and to not shave off more data than necessary. Finally, the neural network is loaded into the vector register efficiently.
The goal is to create a neural network that can run within the constraints of less-intensive hardware, without sacrificing its integrity or accuracy. This not only helps save energy and computing resources but could make deploying these models easier and more accessible.
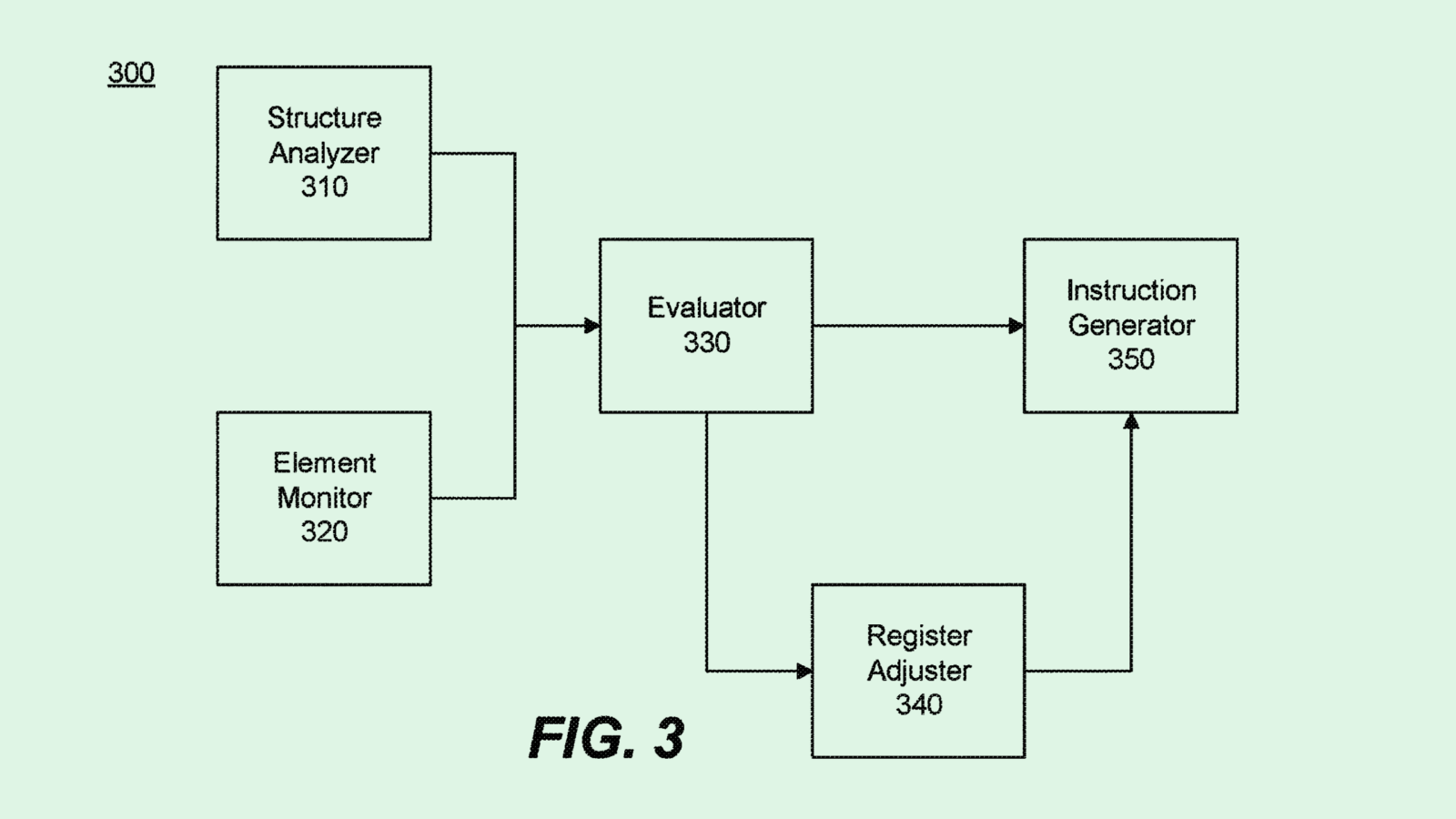
Like every other tech firm, Alibaba has sizable AI ambitions. Eddie Wu, CEO of the e-commerce firm, announced in November its goal to remake itself as “open-technology-platform enterprise to provide infrastructure services for AI innovation and transformation in thousands of industries,” with plans to undergo sweeping restructuring to do so.
The company’s vast cloud services business has given it a leg up, offering AI startups credits to use its computing resources to train AI models in exchange for equity in their companies. With investments in companies like Moonshot, Zhipu, MiniMax, and 01.ai, this model has made way for the company to become a leading AI startup investor in China, according to the Financial Times.
In the most recent quarter, Alibaba’s cloud services sector saw around a 3% year-over-year growth rate, bringing in 25.6 billion yuan, or $3.5 million for the quarter — though AI-related revenue increased “triple-digit year-over-year,” according to Wu. Its core e-commerce business is also leveraging AI for growth with a chatbot for personalized recommendations.
However, its cloud computing division has stiff competition with the likes of Microsoft Azure, Google Cloud, and Amazon AWS. According to CRN, Amazon holds 31% of global cloud services market share, With Microsoft at 25% and Google at 11%. Alibaba, meanwhile, sits at around 4%. Making AI easier to deploy as this patent intends could help lure new developers.
Extra Drops
- Amazon wants to find a throughline. The company filed a patent for “theme detection” within a corpus of documents.
- Block is working on a new way to protect user data. The company filed to patent a card that uses “thermochromic ink,” which changes color when faced with heat to reveal account numbers or other user information.
- Ford wants its cars to help each other out. The automaker is seeking to patent a system for “peer-to-peer” electric vehicle fast charging.
What Else is New?
- Microsoft is set to debut its AI PC this week at its Build developer conference in Seattle.
- Blue Origin successfully completed its first crewed launch since 2022, bringing six tourists into space.
- Curious about the impact of OpenAI’s chief scientist leaving? Wondering what lies ahead for TikTok if it’s sold to a billionaire tech critic? So are we — that’s why we read Semafor Tech, a twice-weekly newsletter that is packed with original reporting, data exploration, witty captions, and must-read tidbits on everything going on in Big Tech. Join 50k CTOs, founders, and tech executives — subscribe for free now.*
* Partner
Patent Drop is written by Nat Rubio-Licht. You can find them on Twitter @natrubio__.
Patent Drop is a publication of The Daily Upside. For any questions or comments, feel free to contact us at patentdrop@thedailyupside.com.