Happy Thursday and welcome to Patent Drop!
Today, a patent from DeepMind may aim to help AI-powered robots improve their reflexes. Plus, Nvidia wants to make text-to-speech more adaptable, and Humane brings dual-screen to your hands.
And a quick programming note: Patent Drop is taking a brief vacation on Monday, April 29, but will be back on Thursday, May 2.
Let’s dive in.
DeepMind’s Risk and Reward
DeepMind wants to make sure its AI models know the world around them.
Google’s AI division filed a patent application for a way to generate a “model of a target environment” based on interactions between an autonomous agent and a “source environment.” DeepMind’s system helps AI models improve decision-making by getting a thorough understanding of several different environments, allowing AI models to be more adaptable.
Controlling an agent, such as a robot or autonomous device, in any environment requires a certain level of uncertainty in decision-making, DeepMind noted. “The agent must navigate the trade-off between learning about reward distributions by exploring the effects of actions versus exploiting the most promising actions based on the current interaction history of the agent with the environment.”
DeepMind’s system selects a series of actions that an autonomous agent can take in its current environment using what’s called an “environment model,” or a simulation of the agent’s surroundings.
Using that simulation, as well as historical data about different actions and interactions that the agent has taken, the environment model is “parameterized,” or tuned to give the agent better situational awareness. Additionally, the system takes in data from several different “source environments,” or places that are different from the agent’s location, which provides diverse data to help the model improve its reflexes and generalize its training.
Finally, this system weighs the risk and reward of every action that a robot can take in a given environment, aiming to come up with the most effective outcome. This process is repeated over time, allowing the agent to essentially learn from its mistakes.
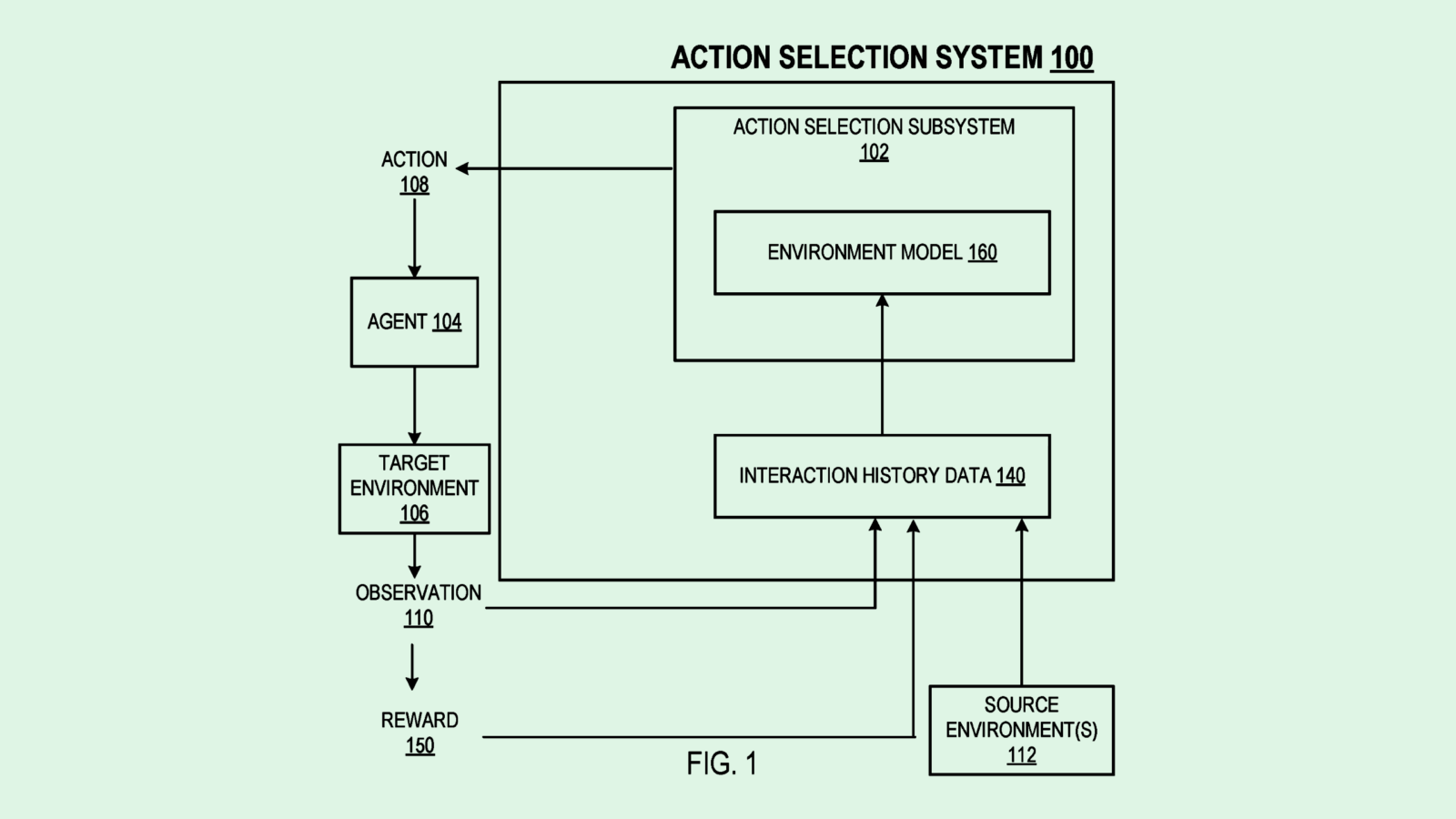
DeepMind’s system essentially aims to let robots explore their options, said Rhonda Dibachi, CEO of Manufacturing-as-a-Service platform HeyScottie. Imagine driving the same route to work every day, but one day it’s blocked by construction. Rather than return to the same route the next day, you explore new routes and learn the trade-offs of different options. “It is a balance between exploring and exploiting,” Dibachi said.
This kind of decisiveness seems obvious to us as humans, but has to be clearly laid out to a robot or autonomous machine, Dibachi noted. These machines are capable of doing a lot of different actions, but often they can only do what they’re pre-programmed for, rather than reacting or using “probabilistic” decision-making, she said.
Breaking past this decision-making barrier is vital in further integrating AI-powered robotics throughout operations like logistics, manufacturing, and construction. As it stands, robots exist behind cages in factory settings to limit their range of motion and prevent safety hazards. DeepMind’s patent, however, may “help them get out of the cage,” said Dibachi.
“If you take those walls down, the dangers multiply infinitely,” said Dibachi. “So what you have to do is go to AI, because it allows the robot to interact with the environment in an open-ended way. This is the next big step.”
Melding AI and robotics has piqued the interest of many big tech firms in recent months. Several big tech firms have gone after AI-powered robotics patents for things like battery life, action planning, and reactivity, and several startups have been hard at work on bringing all-purpose humanoid robots to market.
Aside from this patent, DeepMind has similarly been tinkering with robotics. The company announced in January several advances in its robotics research that help with fast decision-making and environmental navigation. And earlier this month, Ayzaan Wahid, a research engineer at the company, posted a series of videos on X showing off ALOHA Unleashed, a project aiming to push the boundaries of dexterity in autonomous robots. The videos featured robotic arms putting shirts on hangers, tying shoes, replacing machine parts, and tightening gears.
Nvidia Changes the Subject
Nvidia may want to make polymath AI models.
The company seeks to patent a system for “customizing text-to-speech language models” using “adapters” for conversational AI systems. Nvidia’s tech essential aims to create speech models that can understand and adapt to several different users speaking to it.
Training speech models generally takes a large amount of data, and customizing those models requires a large amount of data and training resources for each new user. Additionally, when a model takes in too much information related to several different users, the quality of its output can go down, the filing notes.
Nvidia’s tech aims to avoid resource drain and “overtraining” of these models by training parts of the model, rather than the entire thing, to the specifications of each speaker. Nvidia’s system first gives a model a base training by using a broad dataset filled with reams of speech data.
The next step is adding “adapter layers,” which are meant to be modified to accommodate the information of a specific user. During this part of training, the parameters of the base model are “frozen,” meaning they’re fixed and cannot be changed. New adapter layers can be added whenever new speakers (or groups of speakers) are introduced
The result is a speech model that can essentially know which user is talking to it, as well as any context or information around said user. For example, in a household with several different occupants, a smart speaker trained in this way would be able to differentiate between each one.
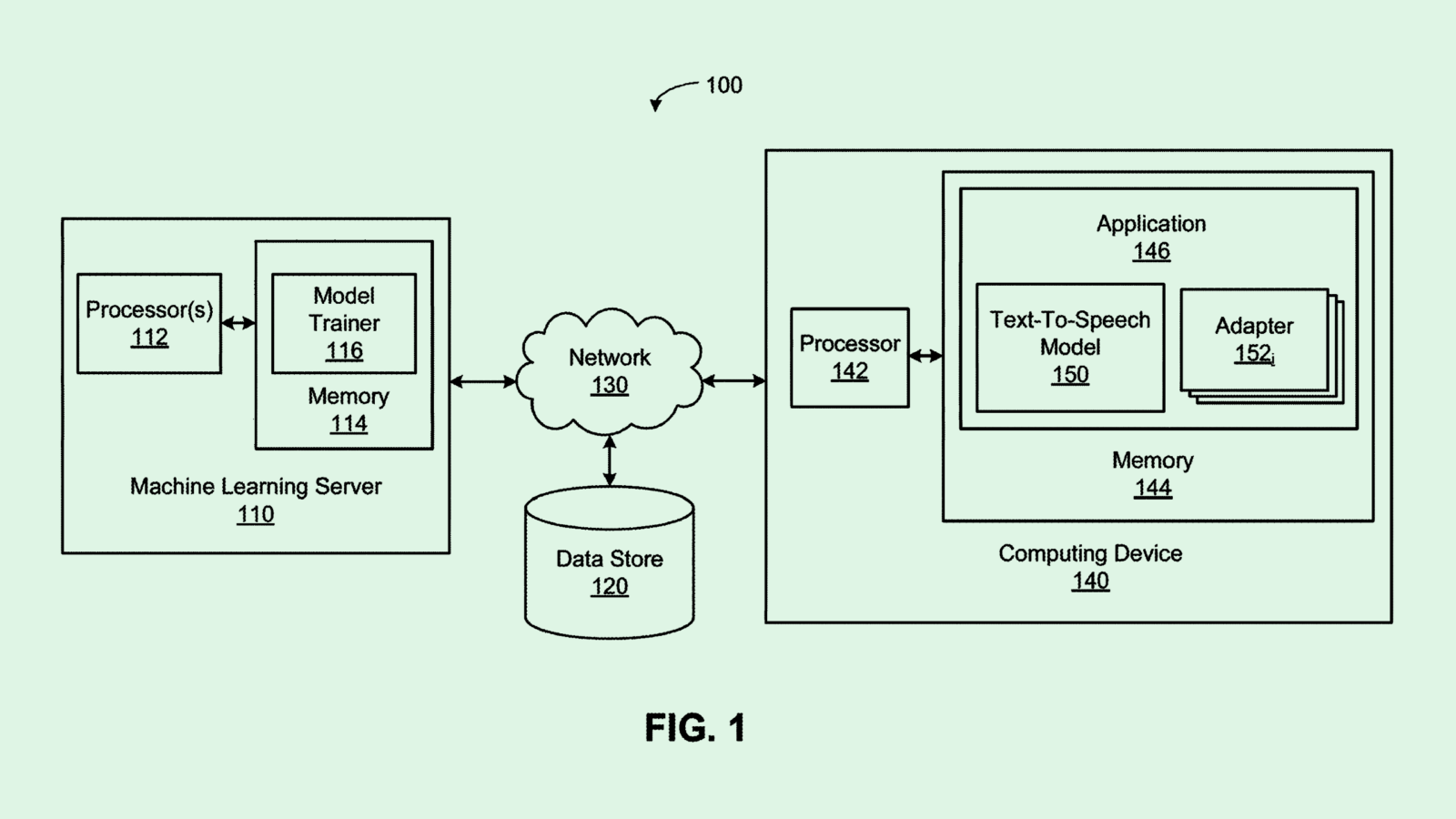
The concepts are quite similar to those being put into use in large language models, said Vinod Iyengar, VP of product and go-to-market at ThirdAI. If a company wants to use a language model for its own purposes, rather than building one itself from scratch, it’s common to use adapters like these to modify outputs.
These layers can be thrown on top of existing architecture without having to do anything to the language model itself. And because massive models take tons of time, resources, and money to create, this can save a smaller company from having to break the bank if they want to integrate AI.
But training in this way comes with sacrifices, Iyengar noted. While you can modify your outputs to a certain extent, it’s still less adaptable than creating your own niche model. Using a generalized model with an extra layer may also prove less accurate to your specific needs. Iyengar likened it to putting lipstick on a pig: “The core problem is not solved” when it comes to how resource-intensive foundational AI training is, so people are looking for workarounds.
“The reality is customization is very hard,” said Iyengar. “You see a lot of tricks to solve the last mile, but you give up some accuracy and some amount of control to maybe get a good enough solution for a lot of these use cases.”
Additionally, adapters like these may get you stuck within a certain development ecosystem – which, for Nvidia, is certainly a boon. While the company makes most of its revenue from chips, its CUDA software ecosystem has everything a developer could want. The goal with this is to make it “sticky,” said Iyengar, offering so much value that its customers become hooked.
Humane’s Ambidextrous AI
Humane may want to occupy both of your hands with its futuristic AI pin.
The company filed a patent application for “hand-specific” laser projections of its virtual interface. As the title of this filing implies, this tech aims to change the user interface based on the hand that the user holds up to the pins projector.
“In the case of the user’s palm, there is very little surface area in which to project a detailed (virtual interface),” Humane said in the filing. “This limited space can limit the number and types of user interactions with the VI, and thus potentially limit the number and types of applications that rely on the VI for input and output.”
Humane’s system would allow users to assign certain operations or applications to each hand. The camera on the device then uses depth detection sensors to determine which hand is in front of it.
Humane noted a few different operations that users could assign to each hand, such as a note-taking app, a calendar, a messaging app, or navigation. For example, if you are texting someone with your left hand, and want to check your calendar without exiting the messaging app, you can assign your calendar to your right hand for easier access.
This would allow a user to interact with these devices in a “more organized, consistent, predictable, and/or intuitive manner,” Humane said, as well as prevent erroneous responses from the device.
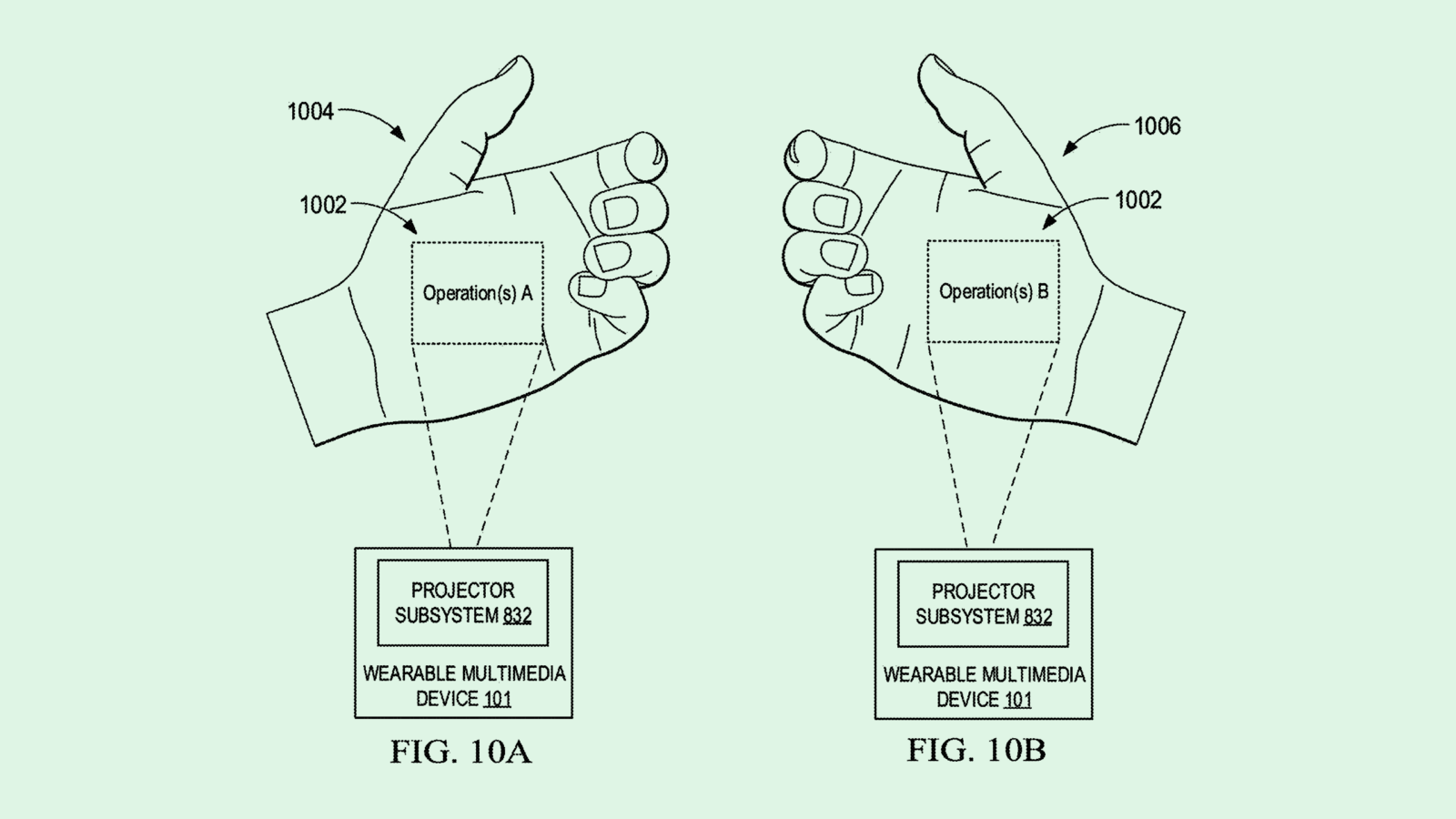
Humane first unveiled its debut device, called the AI pin, in November, promising to change the future of how we communicate with its entirely screenless experience. The company’s ambitious idea attracted hundreds of millions in funding, with backing from tech heavyweights like Microsoft, Tiger Global, Salesforce’s Marc Benioff, and OpenAI’s Sam Altman.
The company has been toiling away at this device since 2018, spending “thousands of hours reimagining and redesigning new types of compute interactions,” CEO and founder Imran Chaudhri said at a TED talk last year. “Why fumble for your phone when you can just hold an object and ask questions about it?” Chaudhri said.
While this sounds great in theory, as the device made its way into the hands of critics some cracks have come to light. With issues like lagging responses, misunderstanding inputs, unreliable battery, and a projector that doesn’t work well in the sunlight, many reviews are finding that the device doesn’t live up to Humane’s promises – especially not at a price tag of $699 with a $24 monthly subscription.
One review in particular, titled ‘The Worst Product I’ve Ever Reviewed… For Now’ by YouTuber Marques Brownlee, stirred up a good deal of social media turbulence, with many concerned that his review had the potential to bury the company. Brownlee, who has 18 million subscribers, noted in the review that the device is a “victim of its future ambition.”
If Humane does want to disrupt the smartphone status quo, the company has a lot of tweaking to do. And while this patent may add a little more usability, it simply brings another palm into the equation when many are hooked on screens.
Extra Drops
- Google wants you to look your virtual best. The company is seeking to patent a method for “puppeteering a remote avatar by facial expressions.”
- JPMorgan Chase wants to help you with feng shui. The bank filed a patent application for “systemizing organizational design using artificial intelligence.”
- Meta wants to know what keeps stealing your attention. The tech firm wants to patent a system for “interference pattern detection” in gaze tracking for extended reality.
What Else is New?
- Meta shares dropped sharply after the company’s earnings call, in which CEO Mark Zuckerberg focused on the company’s expensive long term investments like AI and the metaverse.
- Synthesia, an AI startup backed by Nvidia, debuted “expressive avatars” that can convey human emotions.
- Tesla said that it expects its own 4680 battery cells to be cheaper than those from suppliers by the end of the year. The company announced these cells in 2020 as a low-cost, high range alternative to current options.
Patent Drop is written by Nat Rubio-Licht. You can find them on Twitter @natrubio__.
Patent Drop is a publication of The Daily Upside. For any questions or comments, feel free to contact us at patentdrop@thedailyupside.com.