Happy Monday and welcome to CIO Upside.
Today: The tech industry is split on the timeline for quantum computing, but the applications for finance, infrastructure and cybersecurity could be big – if developers can scale it. Plus: How to avoid AI overreliance and shadow AI; and patents from JPMorgan Chase and Amazon help models unlearn bad habits.
Let’s jump in.
Despite Breakthroughs, Quantum Industry is “Split” On Arrival Timeline
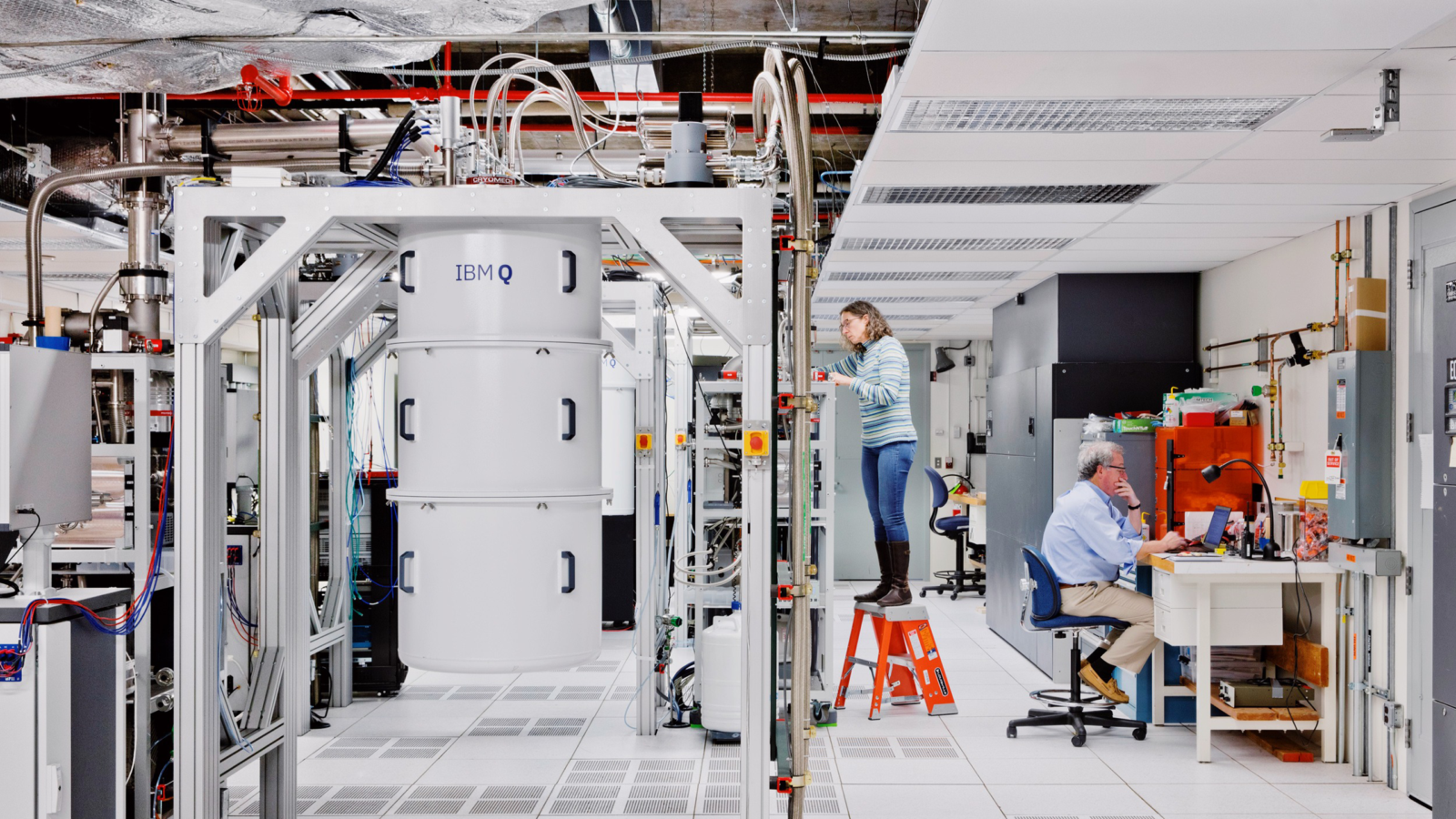
Is practical quantum computing arriving sooner rather than later?
The past several months have been laden with quantum computing breakthroughs and announcements. Google, Amazon and Microsoft all have announced new quantum chips; and JPMorgan Chase claims it’s generated the first truly random numbers on a quantum computer in partnership with Honeywell’s Quantinuum.
With the pace of breakthroughs speeding up, some industry voices see quantum’s onset as a near-term reality. Julian Kelly, Google Quantum AI’s director of hardware, told CNBC last week that we’re only five years out from running practical applications on quantum computers that we can’t on classical ones. Even Nvidia CEO Jensen Huang has walked back naysaying comments in recent weeks on the timeline for useful quantum computers hitting the market.
But despite the excitement, it’s still unclear how close we are to practical, scalable quantum computing that does things that classical computers aren’t capable of, experts told CIO Upside.
“The industry is split,” said Troy Nelson, CTO of LastWall. “Some people aren’t convinced that quantum computing is something we will achieve even in the next 10 or 20 years. But people at the forefront and bleeding edge can see applications fairly soon.”
Quantum computers exist today in some capacity, such as D-Wave’s quantum annealing computer, which is suited specifically for solving optimization problems, said Vaclav Vincalek, tech advisor and founder of Hiswai. However, some “quantum purists” have dismissed D-Wave’s methods, said Vincalek, as they “allow only for a niche type of computing.”
- Plus, even D-Wave’s quantum computer is only a few thousand qubits. Pushing past that and reaching the million-qubit goal that many have their eyes on requires overcoming a number of obstacles, said Vincalek. One of the biggest barriers is noise, or vibrations, electrical interference or really any environmental factor that can knock these devices out of superposition.
- “I think that five years is still hugely optimistic,” said Vincalek. “In order to get to something useful … you need tens of thousands, if not hundreds of thousands, of qubits. Scaling is still a major challenge.”
As it stands, the quantum computing market size is relatively small, sitting at $1.07 billion in 2024, according to the State of the Global Quantum Industry report, released by QED-C in March. But given quantum’s capability to impact cryptography, which has cybersecurity effects across critical infrastructure like supply chains, logistics and defense, the market potential could be “immeasurable,” said Nelson.
“When we get there, it’ll be a breakthrough for humanity, much like the advent of the computer itself,” he said.
However, when this tech does make it to the mainstream, there’s one industry in particular that has the “most to lose and most to gain,” said Nelson: finance. On one end, Wall Street firms can take advantage of this tech for financial risk-modeling and optimal portfolio planning. And on the other, these firms need to be “ahead of the curve” from a defensive point of view, said Nelson.
“If we’re talking about a cryptographically relevant quantum computer that can break modern (security protocols), that gives attackers the ability to open up everything that’s encrypted and take what they want,” said Nelson.
How to Keep Your Workforce from Overusing AI
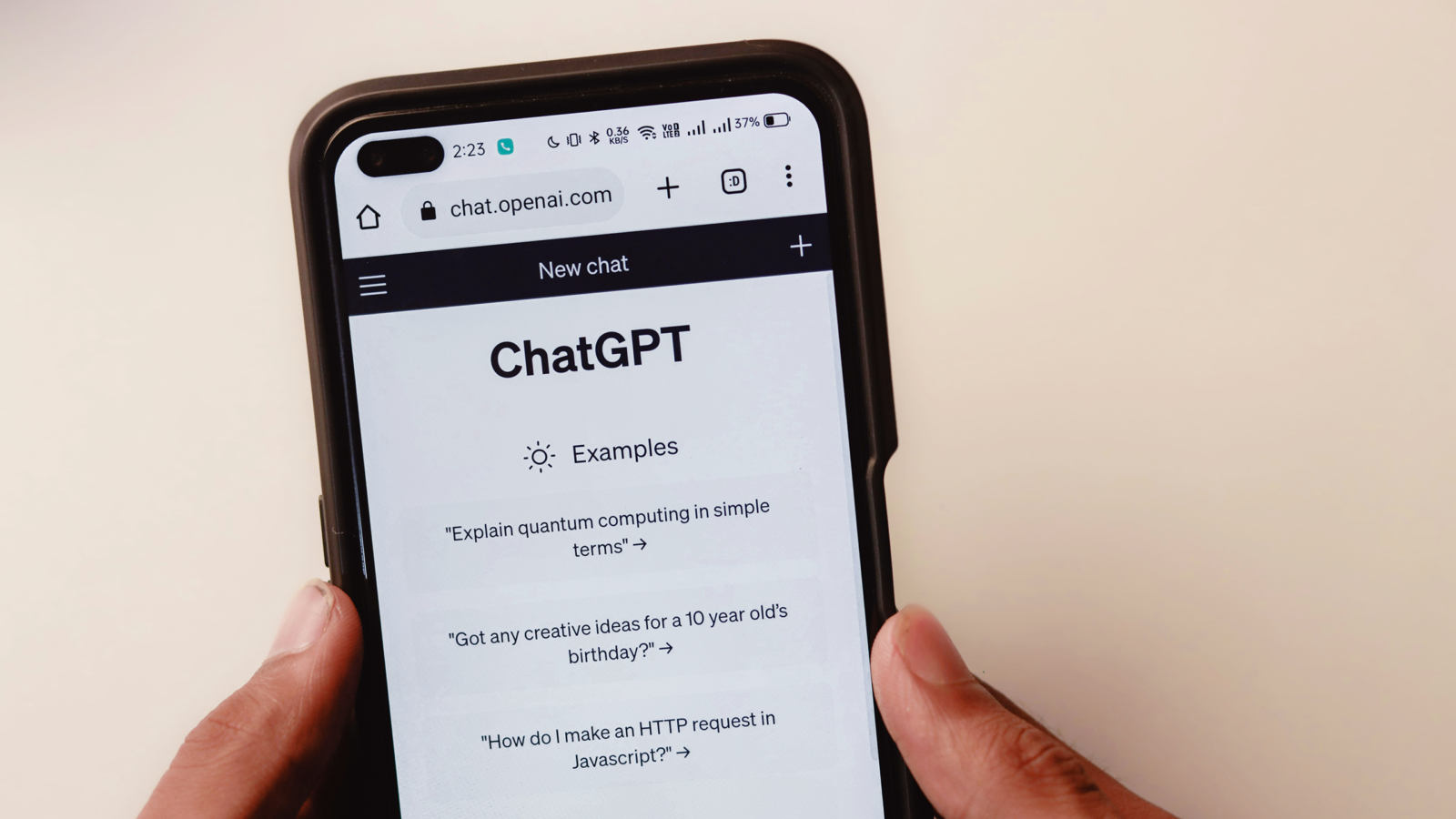
Overreliance on AI can lead to far more problems than simply making your workforce less industrious.
AI can be addictive, and with enterprises adopting more AI tools and integrating agentic capabilities, the question of where to draw the line is on the minds of many CIOs. Lacking a clear AI policy and proper workforce education could lead to the use of shadow AI, or AI tools unsanctioned by employers, exposing employers to security risks and other liabilities.
“Using AI tools on a regular basis, it can be habit-forming,” said Zac Engler, CEO of AI consultancy Bodhi AI. “There definitely is a danger of over-dependence on these systems.”
Though AI can do a lot for us, it still faces issues with data security and hallucination if used improperly. And whether or not you’re aware of it, your employees are probably using AI.
- A study of 6,000 knowledge workers by AI firm Perplexity found that 75% use AI daily, and around half are engaging in shadow AI activity. Around 46% said they’d continue to use AI even if their organizations banned it outright.
- Researchers from Carnegie Mellon University and Microsoft found that the overuse of AI could hinder critical thinking potential.
Avoiding the pitfalls of overreliance and the use of shadow AI is all about having a clear cut strategy, said Engler. Teaching employees when they should and shouldn’t use AI is critical as more of these tools are adopted. “We’re essentially shepherds of the machine,” he said. “No shepherd should ever let the sheep decide where to go.”
“The real risk isn’t necessarily the use of AI, it’s the overreliance without a strategy,” Engler added. “Overreliance tends to happen in organizations when they treat AI as the decision-maker rather than a decision-support tool.”
So how can you find that middle ground? The first step is figuring out where AI isn’t necessary, said Engler. Look for places where humans should remain in the loop, such as critical decision points, versus the tedious and low-stakes tasks that don’t need a human hand. If you’re worried about hallucination, asking your AI systems for “confidence thresholds,” or how confident the model is in its work, could help mitigate accuracy issues, he said.
“The sooner you can figure out how to ride that wave of this continually improving and continually evolving system, the sooner you’re going to be able to succeed,” said Engler.
JPMorgan Chase, Amazon Seek Patents to Make AI Unlearn Bad Habits
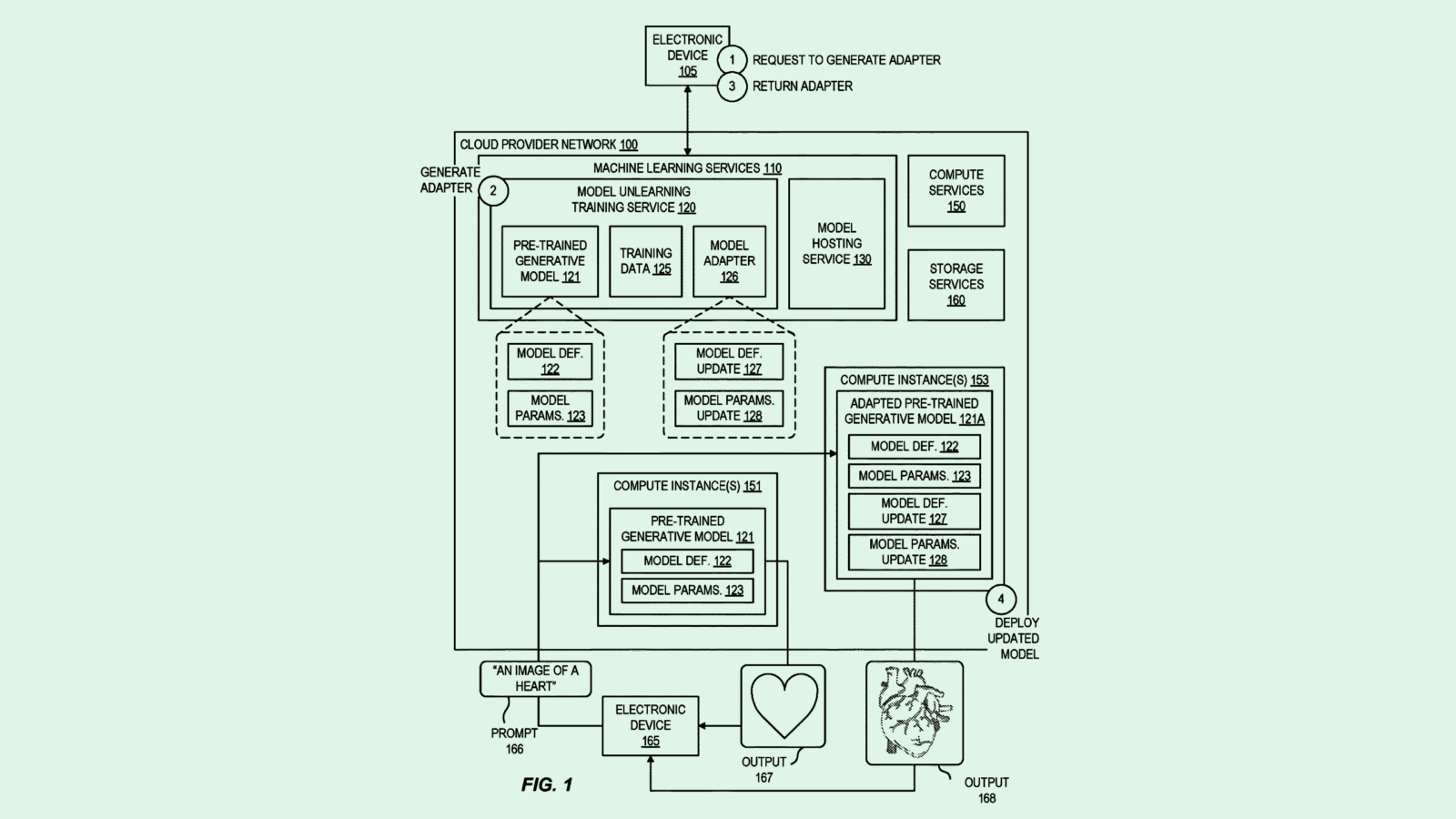
AI models tend to pick up bad habits from their environments, and some firms are working on ways to help systems “unlearn” them.
JPMorgan Chase and Amazon both filed patents for “unlearning” in generative models, tech that could help developers avoid having to entirely retrain models as well as strengthen AI guardrails against data security slipups.
To start, JPMorgan Chase is seeking to patent “machine unlearning in generative models.” This essentially feeds a model a “forget dataset,” which trains it to treat certain data points as meaningless noise. The model is also fed a “retain dataset,” to help it hold onto relevant knowledge.
Similarly, Amazon filed an application to patent “unlearning in pre-trained generative machine learning models.” Amazon’s tech helps models unlearn specific concepts or data by feeding them “negative prompts,” or data they should forget, and “positive prompts,” or data they should remember. Instead of retraining the whole model, the system uses these prompts to create an “adapter,” or a filter that adjusts how the model responds.
Generative models are essentially parrots: If they’re approached with the just the right prompts, it’s possible to get them to reveal the data they were trained on. These techniques are just a few of several that tech firms are eyeing to get AI’s data security problem under control. Google, Microsoft, Intel and others have all sought patents for ways to lock down AI’s training data.
But with the rate at which enterprises are seeking to adopt AI, especially with the rise of autonomous AI agents, there’s a constant push and pull between innovation and security. To avoid slipups that can cause both financial and reputational damage, one has to come before the other.
Extra Upside
- DeepSeek Competition: Alibaba released an open source AI model for developing cost-effective agents.
- Board Shake-up: Three members of Intel’s board of directors announced that they’re retiring in May.
- Healthcare Hack: Hackers breached Oracle’s systems and stole patient data, aiming to extort medical providers.
CIO Upside is written by Nat Rubio-Licht. You can find them on X @natrubio__.
CIO Upside is a publication of The Daily Upside. For any questions or comments, feel free to contact us at team@cio.thedailyupside.com.