Happy Thursday and welcome to CIO Upside.
Today: As enterprises scrap AI implementations, one expert discusses how firms can have better AI sticktoitiveness. Plus: What Intel’s comeback could mean for the semiconductor industry; and IBM’s patent could make LLMs more secure.
Let’s jump in.
Businesses Are Scrapping AI Projects. Here’s How to Find What Sticks
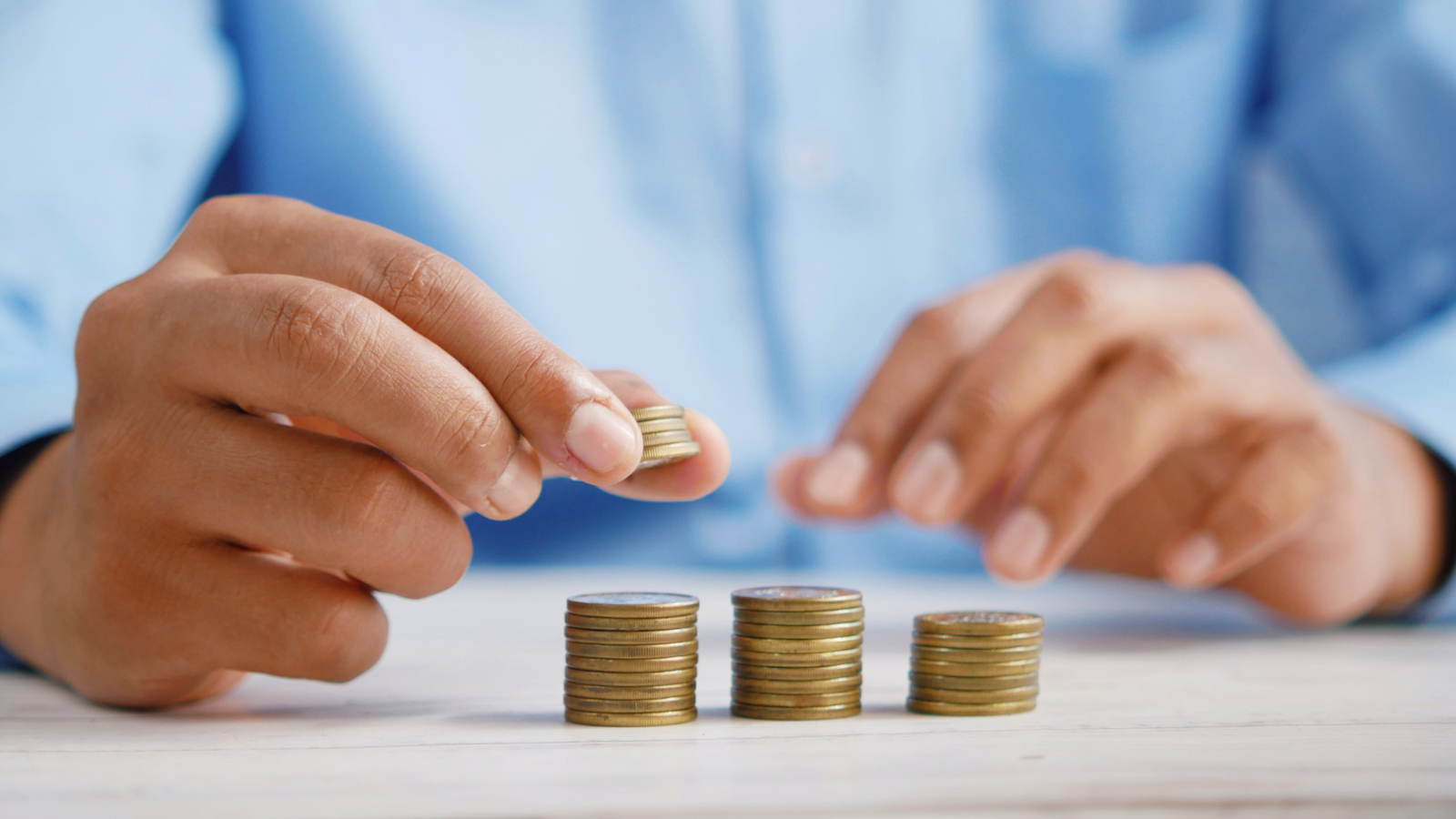
Enterprises want to take advantage of AI, but figuring out what applications stick is taking a lot of trial and error.
That lack of clarity is illustrated in the accelerating rate at which businesses are dropping AI projects: According to S&P Global Market Intelligence, the number of companies that scrapped AI initiatives grew from 17% in 2023 to 42% in 2024, with the average enterprise cutting 46% of AI proofs-of-concept.
There are a number of reasons that these projects don’t make it out of the “infancy phase,” said Bob Rogers, chief product and technology officer of Oii.ai and co-founder of BeeKeeper AI. For one, a lot of companies are in a discovery phase with AI, in which they’re “throwing spaghetti at the wall and seeing what sticks,” he said.
- Many find that scaling projects outside of small environments or datasets can be quite tricky, said Rogers. “They hit some fairly serious snags with the scruffiness of real life data.”
- Factors like cost, privacy and security issues similarly put pressure on CIOs to be stringent about which AI projects they scale and which ones they scrap, he said.
- Plus, hiring AI teams to actually build and manage these projects is a challenge in and of itself, said Rogers.
That leads many to the “build or buy” equation, said Rogers. While building an internal model that works only on your enterprise’s data can make it more niche, the difficulty of scaling may lead these firms “more in the buy direction,” he said, choosing models or AI integrations offered by outside sources. “The maturity of offerings coming from outside is going to be better,” said Rogers.
Internal projects need to make “that leap of making security, reliability, integration with other tools industrial-strength,” Rogers said.
So how can enterprises have better AI sticktoitiveness? One way is to set benchmarks from the start of a project, said Rogers. If you don’t know what exact metrics your AI project can improve, then figuring out whether or not a project will see returns in the long run is all the more difficult.
“Benchmarking is a critical component,” said Rogers. “If you don’t have as part of your plan a benchmark of what it is that you’re really improving … if you hit some tough sledding, it’s really, really hard to keep the momentum.”
Having the foresight to account for security problems and the “weirdnesses” of real-life data can also help enterprises get their AI projects out of the sandbox, Rogers said. “None of the AI projects that are hypothetically thrown away are doomed to failure for sure,” he said.
“Quite often there’s actually an opportunity to move forward,” said Rogers. “But the wherewithal of an organization to see through that noise and get to the next level is very challenging.”
AI Won’t Run Your Business, But It Can Optimize It
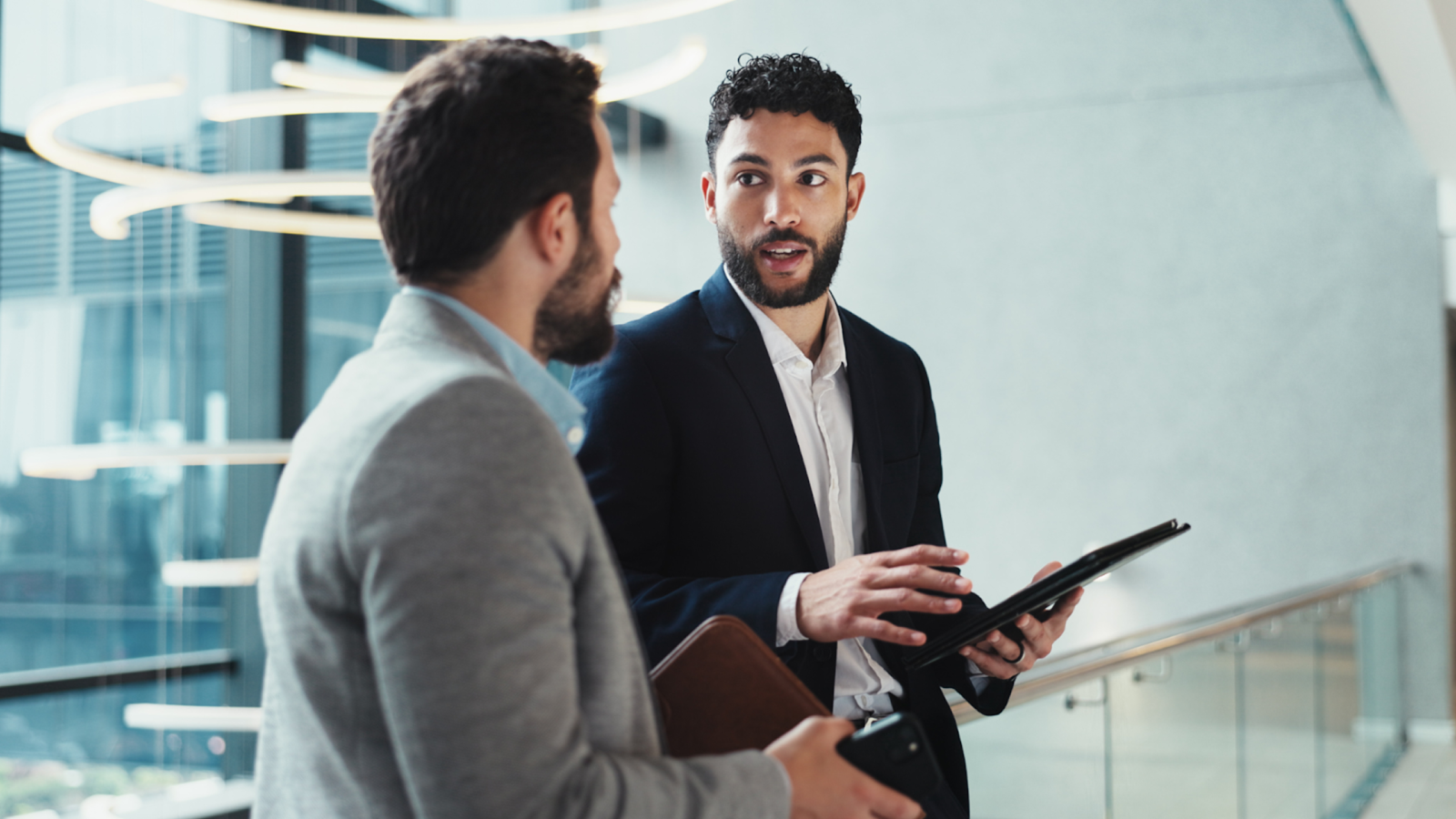
AI-driven companies outperform competitors by 5X, yet most businesses still struggle to harness its full potential (Accenture). The major players in tech — Netflix, Amazon, Amex — are using AI predictive analytics to refine their strategies, optimize performance, and stay ahead… And clearly, it’s working. But AI isn’t just for the tech giants.
The key is not in blindly trusting Chat GPT to make your business decisions, but instead knowing when to trust AI, when to test it, and when to take the human-led approach.
Oracle NetSuite’s “Optimize Your KPIs With AI” field guide offers a practical framework for using AI to enhance forecasting, refine KPIs, and turn data into actionable insights, without losing control.
Nail your KPIs this quarter — Download “Optimize Your KPIs With AI” now.
Can Intel Get its Groove Back?
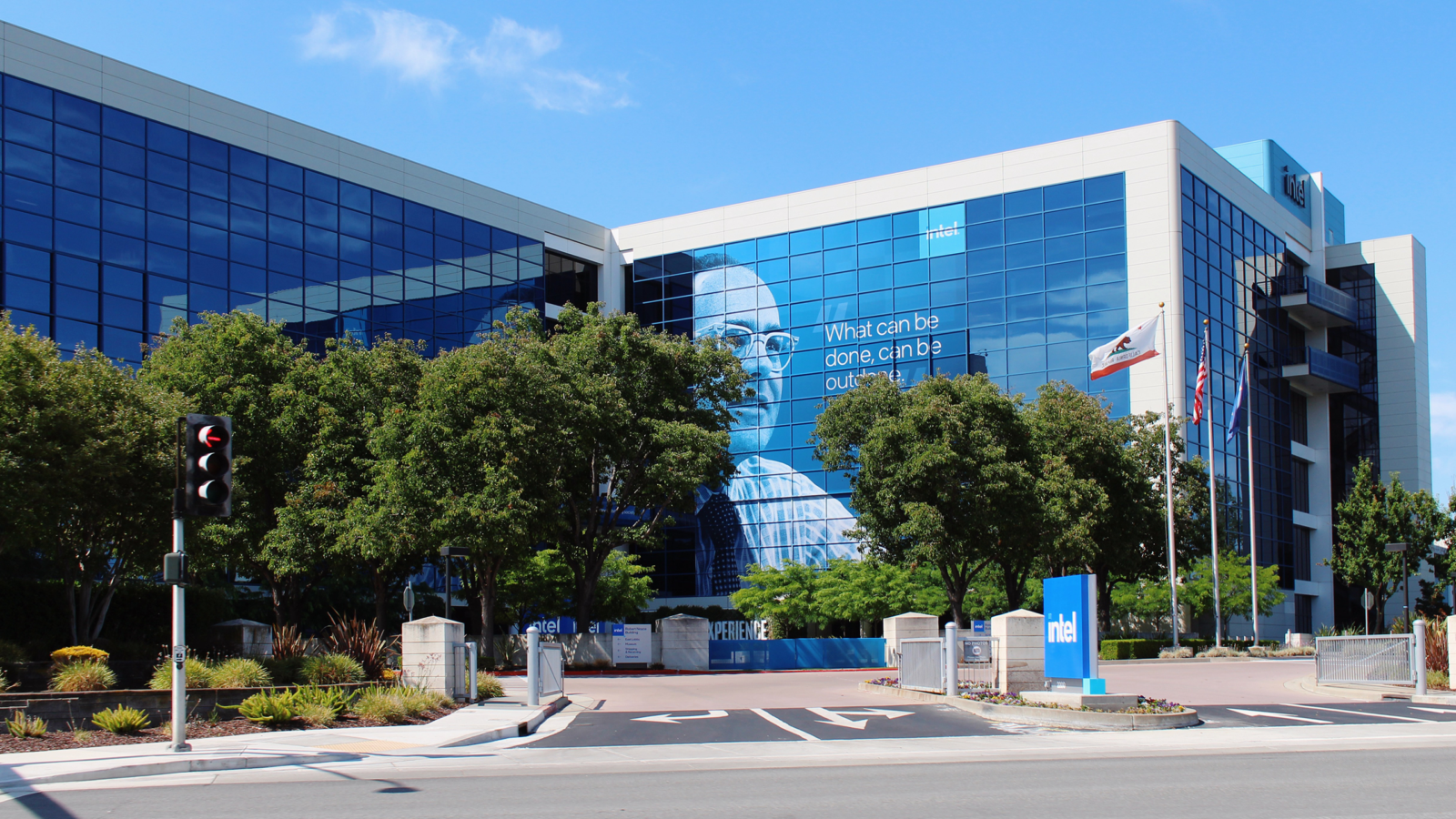
Intel, which has lost some of its once near-universal allure to investors, may be getting a facelift.
While the beleaguered chip firm has struggled in the booming AI chip market, incoming CEO Lip-Bu Tan is considering big changes to its manufacturing and AI strategies, Reuters reported earlier this week.
The plans reportedly include cutting staff from the middle management layer of the business; improving its manufacturing arm, Intel Foundry, by garnering new customers including Microsoft and Amazon; and producing AI server chips as well as boosting AI units like software, robotics and models, according to Reuters.
Building up its foundry business will likely be a major growth driver for Intel, said Dinakar Munagala, CEO and co-founder of AI chip company Blaize. “With the economics of foundries … you have to keep it 98% busy in order to cover the investment, and AI is going to be one of the largest growth drivers for the foundry business,” said Munagala.
- Gaining more customers and building up these foundries’ AI chip production could be key in “competing against TSMC in a meaningful way,” he added.
- Munagala said that edge AI in particular, or the deployment of AI in the physical world, could be a big area of opportunity for the firm: “Smart cities, defense, education, healthcare — purpose-built silicon for the edge — I think can be a massive growth driver for Intel.”
But making a comeback isn’t an overnight process, said Munagala. Intel has spent $50 billion on its foundry business in the last several years, and announced plans to spin out the unit last year. Because of the massive capital investments that go into foundries, it takes a long time to see the payoff, he said.
“It’s a three, four, five years-plus kind of time horizon before you start seeing any results,” said Munagala. “It’s a slow-moving train.”
IBM Patent Keeps Users from Oversharing With AI Models
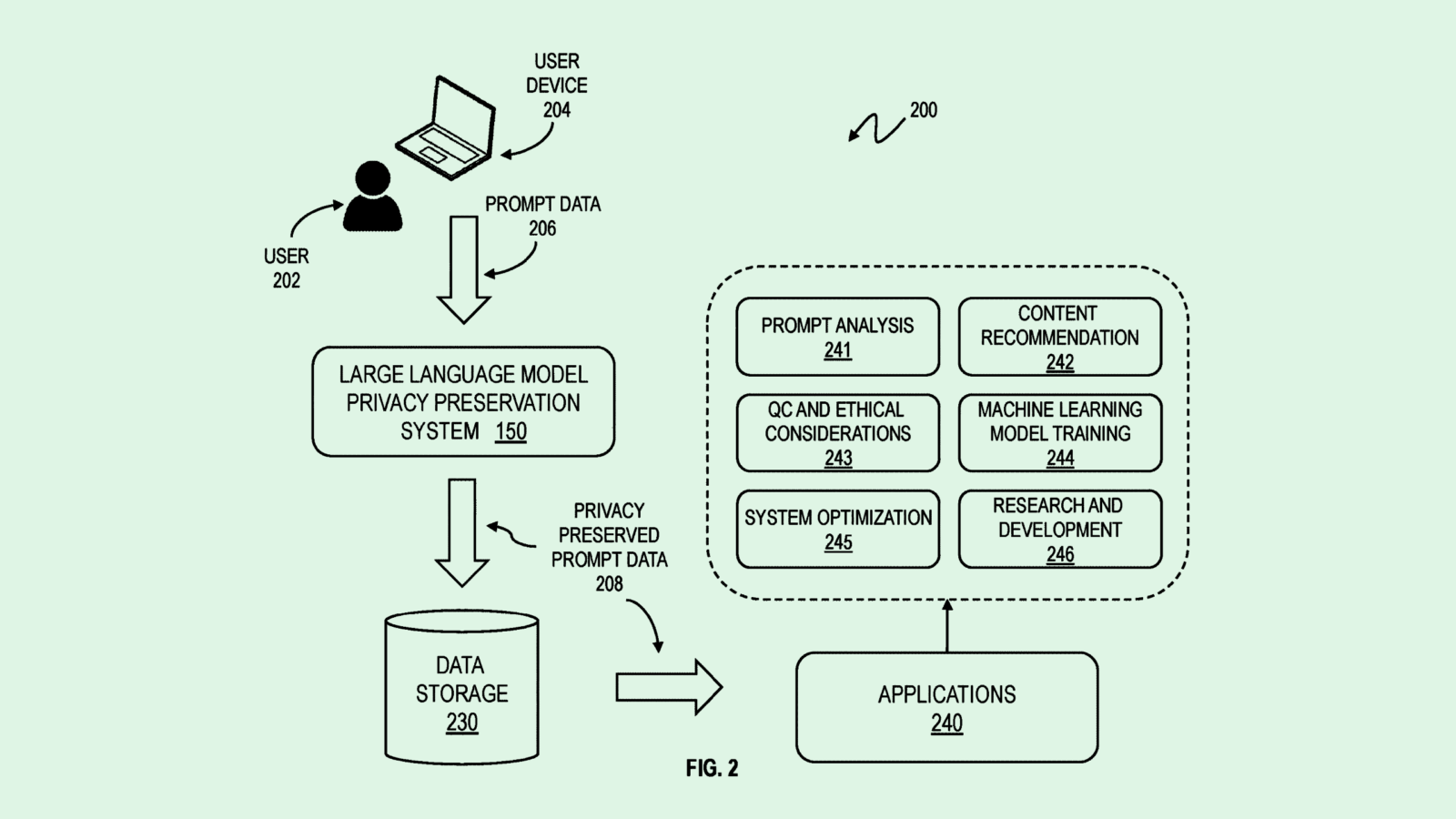
With AI still besieged by security issues, IBM is examining ways to keep personal data from being put into models in the first place.
The company filed a patent application for a “large language model privacy preservation system” that would monitor prompt inputs for sensitive data, then modify them before they are processed by the model if that data is present.
“Storing the prompt data could potentially reveal sensitive information about users and reduce user trust in the large language model,” IBM said in the filing. “Existing solutions, such as data compression, encryption, federated learning, and differential privacy present tradeoffs in efficiency, accuracy, and usability.”
The system scans user inputs to generative AI models in search of personally identifiable information, like names, emails or phone numbers. Along with catching that data, the system classifies the prompt into a relevant category, helping the model understand its intent without revealing personal data.
IBM’s tech then substitutes the personalized information with generalized placeholders, and stores only the anonymized version of the user prompt for training.
Lots of companies have sought solutions for AI models’ ever-present data security risks. Microsoft previously sought to patent tech that prevents AI prompt injection attacks; Google filed a patent application for ways to anonymize large-scale datasets; and Intel wants to patent a way to verify that an AI model hasn’t been tampered with after deployment.
And AI integrations are causing many cybersecurity teams to rethink their strategies: A report released Monday from Bedrock Security found that nine in 10 cybersecurity professionals reported evolving job responsibilities in the past year, largely relating to AI and data governance.
The problem, however, is that the speed to AI development is rapidly outpacing the ability to monitor and fix its security issues. With this rapid uptick, the onus for AI governance and data security best practices has largely fallen on the enterprises that use them, rather than the model developers themselves.
A patent like IBM’s could prevent data missteps at organizations that are still figuring out where AI governance and education fits in, catching private information before it makes its way into a model at all.
Extra Upside
- The Wiz and I: Google is buying cybersecurity startup Wiz for $32 billion, the tech giant’s largest deal yet.
- Chip Crazy: Nvidia announced new AI chips at its GTC conference this week – Blackwell Ultra, shipping later this year, and Vera Rubin, shipping in 2026.
- It’s Electric: Abu Dhabi-based wealth fund ADQ and U.S.-based Energy Capital Partners will invest over $25 billion in powering data centers for AI.
CIO Upside is written by Nat Rubio-Licht. You can find them on X @natrubio__.
CIO Upside is a publication of The Daily Upside. For any questions or comments, feel free to contact us at team@cio.thedailyupside.com.