Happy Monday and welcome to CIO Upside.
Today: CIO Upside sat down with Kurt Muehmel, head of AI strategy at AI firm Dataiku, to discuss the dos and don’ts of deploying autonomous AI agents in your business – and why waiting on agents could cost you.
Let’s jump in.
Want to Deploy AI Agents? Take an ‘Incremental Approach’
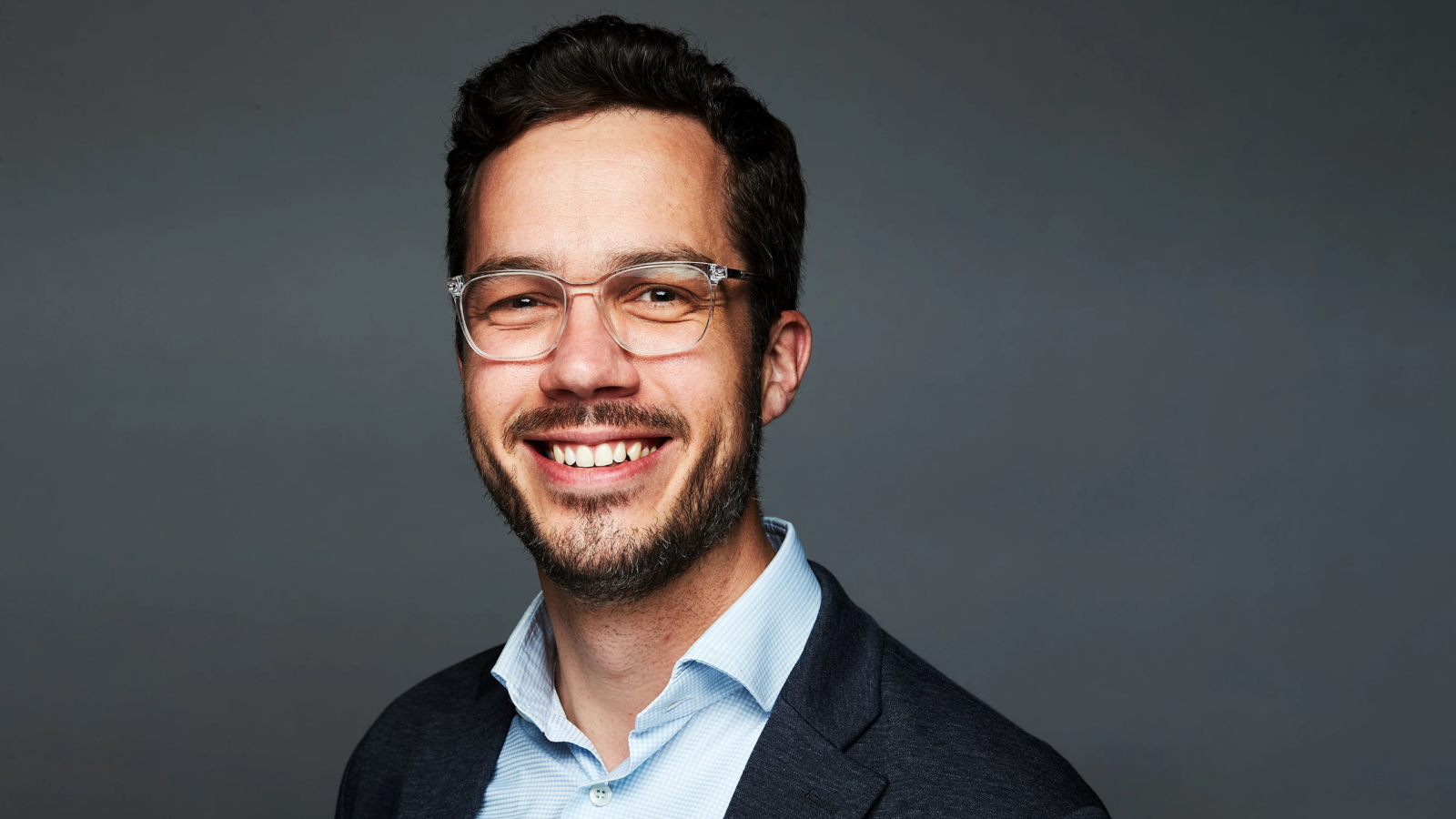
With AI agents, you don’t need to do everything all at once.
Despite the current hype around deploying autonomous AI agents in every facet of your business, the cost and data security risks may make the prospect seem daunting. Waiting, however, may pose an even bigger threat, leaving your enterprise at a steep disadvantage.
“The risk of waiting is that you’re going to be giving your competitors the time to figure this out for themselves, while you’re sitting back and watching from the sidelines,” said Kurt Muehmel, head of AI strategy at AI firm Dataiku. “The costs are potentially very high, and the benefits are very low.”
In an interview with CIO Upside, Muehmel discussed the right way to deploy AI agents, enterprises’ common agentic missteps, and how to keep the tech from going haywire. The conversation has been edited and condensed for clarity.
You’ve been with Dataiku since it was a 20-person company. How has the company’s approach to AI changed since you started?
I would say it’s been extremely stable, despite all the changes around it. The product is miles beyond where it was a decade ago, but always the vision was about making it so that organizations … can use the best technology that there is to get more value out of their data.
Big data analytics was kind of the hot thing, then it became machine learning and developing predictive models that could then be managed and deployed within the enterprise. And then, of course, generative AI, and now agents. So really, it’s been a matter of keeping our eyes out for what is that next technology that’s going to be on the horizon, understanding how our customers are going to want to use it.
In the age of agents, what do you think enterprises are getting right – or wrong – about their strategies?
Let’s start with the positive. The fact that they are interested is a good thing. Sometimes organizations can be too dismissive of things as just a fad. I don’t believe that that’s the case with agents. Agents have a huge benefit for the enterprise, and we’re only beginning to see what that may be.
A lot of headlines are talking about how companies are going to be replacing this department, or that function with agents. That’s not the case in a lot of the businesses that we’re working with. They’re looking at taking existing assets, existing chatbots, existing machine learning models, existing data analytics, and enhancing those with agentic capabilities.
I think that’s important because, given the fact that agents can be … unpredictable by definition, they potentially could do any number of things that present a lot of risk to organizations. The science of developing and testing agents is not fully evolved yet, so it’s appropriate that organizations are taking an incremental approach, while still also being ready to go fast once those best practices are established within the ecosystem of developing agents.
We’re really encouraging them to consider how they’re going to build differentiated agents for themselves. How do they build and deploy that first one if they’ve not done it before? And then, of course, they scale that practice once they get past that first one.
How should enterprises decide where that first agent should be deployed?
A lot of our customers are looking, first and foremost, at internal use cases, so not necessarily something customer-facing. Not an agent which is going to serve your customers through your retail website, but rather an agent which is going to serve your sales associates in your stores. I think that that’s a good place to start: looking for internal use cases where you can make sure that there’s a human in the loop.
Areas that are document-heavy and require working across a lot of unstructured data are very promising. I would tend to focus on areas that can benefit from a lot of automation.
What risks do AI agents present?
First, there’s the fundamental risk that an agent just doesn’t do anything useful for the company. You spend a lot of time, a lot of money, and then the thing is not actually useful for its intended purpose. So that’s the risk of failure.
Second, given the fact that we define an agent as involving multiple steps without interaction with a human, one of the risks there is that it can quickly spiral out of control. It starts generating these very large prompts that start generating these very large outputs. From a cost perspective, that can all of a sudden become very expensive. So there is definitely a cost and budget risk.
Finally, there are reputational and legal risks. Again, we’re talking about systems that can generate text arbitrarily. If they do not have any guardrails, if there are no content filters on them, if there are not systems put in place to appropriately control these agents, then an organization deploying them could find themselves on the wrong side of various legislation, and also on the wrong side of human decency.
What can enterprises do to mitigate those risks and keep agents from losing control in the first place?
An agent can be defined either very broadly or very narrowly. If we were to widely scope an agent, that might result in a system which is perhaps accessing data or systems that it should not access, and which is not correctly solving the business problem, because it’s coming up with what it thinks is its own good idea.
So instead, what organizations must be doing is scoping their agents appropriately. Since we’re at the beginning of the development of reliable agents in the enterprise, I would argue that those should be narrowly scoped to begin with. It means that the way that you’re defining the business problem that this agent should be solving is very focused, and you instruct the agent to behave that way by writing prompts in a very specific way that ensures that it’s only going to do certain things.
What are the costs and benefits of waiting until the “kinks” of AI agents are worked out before deploying?
The statistical problems associated with building an agent, that’s all OpenAI and the model developers who are handling those. You don’t need to develop a new LLM to build a good agent. What you need to define is a really strong business process that can be partially automated by a system like this. And so a lot of the specific knowledge about how to build and deploy agents – yes, there will be general best practices – a lot of it is going to be specific to any one business.
I think there’s really a lot of risk and costs associated with waiting. There’s actually a lot of benefit in trying, making a few mistakes and trying again on narrowly scoped, safe use cases. Then within six months, 12 months, 18 months, your organization is really able to seize upon this technology.
What advice do you have for enterprises seeking to deploy AI agents?
One is don’t just think you can buy your way out of this problem. You’re going to need to build agents of your own in order to have those differentiated capabilities that nobody else has, that are actually getting you beyond what your competitors are doing, rather than just keeping up with them.
Two is, when you do set about building these agents, think beyond building just the first one, and think how you’re going to manage a fleet of agents in the future. We’re in this really interesting time where … what happened over years for machine learning is happening in months for agents. We’re in a really accelerated timeline, so organizations both need to be thinking about building their first one, and also scaling out from there. And probably won’t be surprised that our recommendation will then be to consider a platform rather than trying to do it yourself.
How does Dataiku approach agent building?
Our general approach, especially in the space of agents, is to provide three things. One is connectivity, so that you can connect your agents to all of the different LLMs and service providers out there, but also connectivity to data, data sources and compute resources. Then there’s a creation layer for all those different types of builders, people who want a visual interface, as well as people who prefer a coding interface.
And then a control layer. Everything in terms of cost, content, safety controls, performance measurement – all of that is provided by the platform. And all of that is wrapped in a governance cocoon to make sure that everything that’s happening is well governed.
Our belief on agents is really focusing on ensuring that companies understand that they … have the means to build their own agents themselves without having to do all the plumbing underneath it. I think that’s really the advantage of building agents in a platform – the standardization and modularity it provides.
Extra Upside
- Meta Models: Meta announced the release of its Llama 4 AI models, claiming the models are natively multimodal.
- ChatGPT Overseas: Though adoption of OpenAI’s ChatGPT is soaring in India, it’s struggling to monetize in the country compared to the U.S.
- Mindstream Is Your Daily AI Advantage In A Rapidly Evolving Landscape. Join 200,000+ industry leaders, innovators, and professionals who rely on our concise newsletter for the AI insights that matter. Join today.*
* Partner
CIO Upside is written by Nat Rubio-Licht. You can find them on X @natrubio__.
CIO Upside is a publication of The Daily Upside. For any questions or comments, feel free to contact us at team@cio.thedailyupside.com.