Happy Thursday and welcome to CIO Upside.
Today: With new AI models hitting the market left and right, how can enterprises pick what works best for them? Plus: Protecting your operational tech stack may be easier than you think; and Microsoft’s patents keep its data healthy.
Let’s jump in.
With New Models Every Day, Enterprise AI Battle is ‘Fair Game’
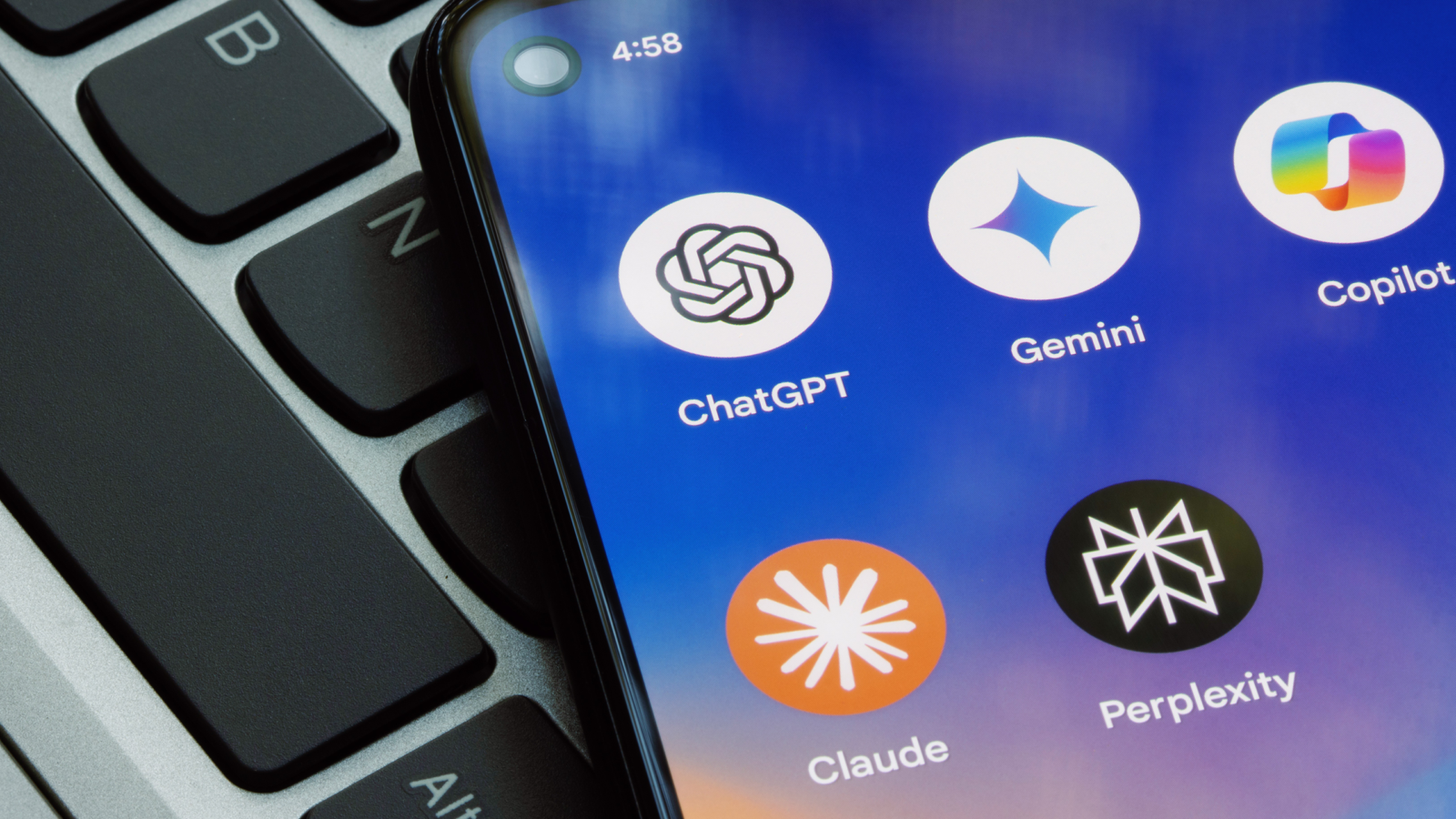
With tech giants pushing bigger and better AI models at a near constant rate, it’s natural to feel a bit of model fatigue.
Google, Meta and OpenAI all added to their families of AI models last week, broadening a field of choices from AI firms big and small that have debuted in recent months. While the models perform differently, their developers are often chasing similar benchmarks and a similar market, experts told CIO Upside.
So how do you choose which family will work best for your business? There are “five dimensions” that enterprises should consider, said Martin Bufi, research director at Info-Tech Research Group: Intelligence, output, speed, latency and price.
Many consider factors such as the best “bang for my buck” when it comes to cost, operational speed and robustness for their specific use cases, said Bufi. And because some models are better suited for different tasks, “it’s not really just who has the biggest, the baddest and the most intelligent model,” he added.
“The other thing to consider is that there is no one model that does everything, when we think about what enterprise means,” said Bufi. For example, while one model may work best for front-end or chatbot operation, another may work better for workflow or task automation.
And with the risk of autonomous AI, agentic processing capabilities are often top of mind for enterprises, said Brian Sathianathan, CTO and co-founder of Iterate.ai. “In order for an LLM to be successful within an enterprise, the model has to execute agentic processes really well.”
For a long time, OpenAI’s models, in partnership with Microsoft’s Azure cloud suite, were the “de facto standard” that enterprises leaned toward, said Bufi. But as enterprises have started running into limitations, said Bufi, some have sought alternatives.
One of those limitations is OpenAI’s context window, or the maximum amount of text that a model can take in at once, he said. Short windows “can be potentially problematic with dealing with long-winded conversations or background context,” he said:
- Google’s context window, meanwhile, is “unmatched” at up to 2 million tokens, Bufi noted. That, in combination with the company’s in-house hardware and search prowess has given Google a boost that’s made scaling in the market far easier, he said.
- Anthropic’s Claude models are another contender for enterprise AI darling, said Bufi. They are particularly talented at technical tasks, such as coding, and are often preferred by software developers.
- Open source models are also “catching up,” said Bufi. DeepSeek’s R-1, Alibaba’s Qwen series, and Meta’s Llama models (despite the company fudging its AI benchmarks) could offer more affordable alternatives to their closed source competitors.
With all these different factors to consider, “the enterprise battle is fair game,” said Sathianathan. “There is a lot of room left for algorithmic innovation … on the business side, from a cloud perspective, a few people will take over everything.”
Who those winners will be, however, has yet to be revealed.
Why CIOs Need Full Visibility into ‘Opaque’ Operational Tech
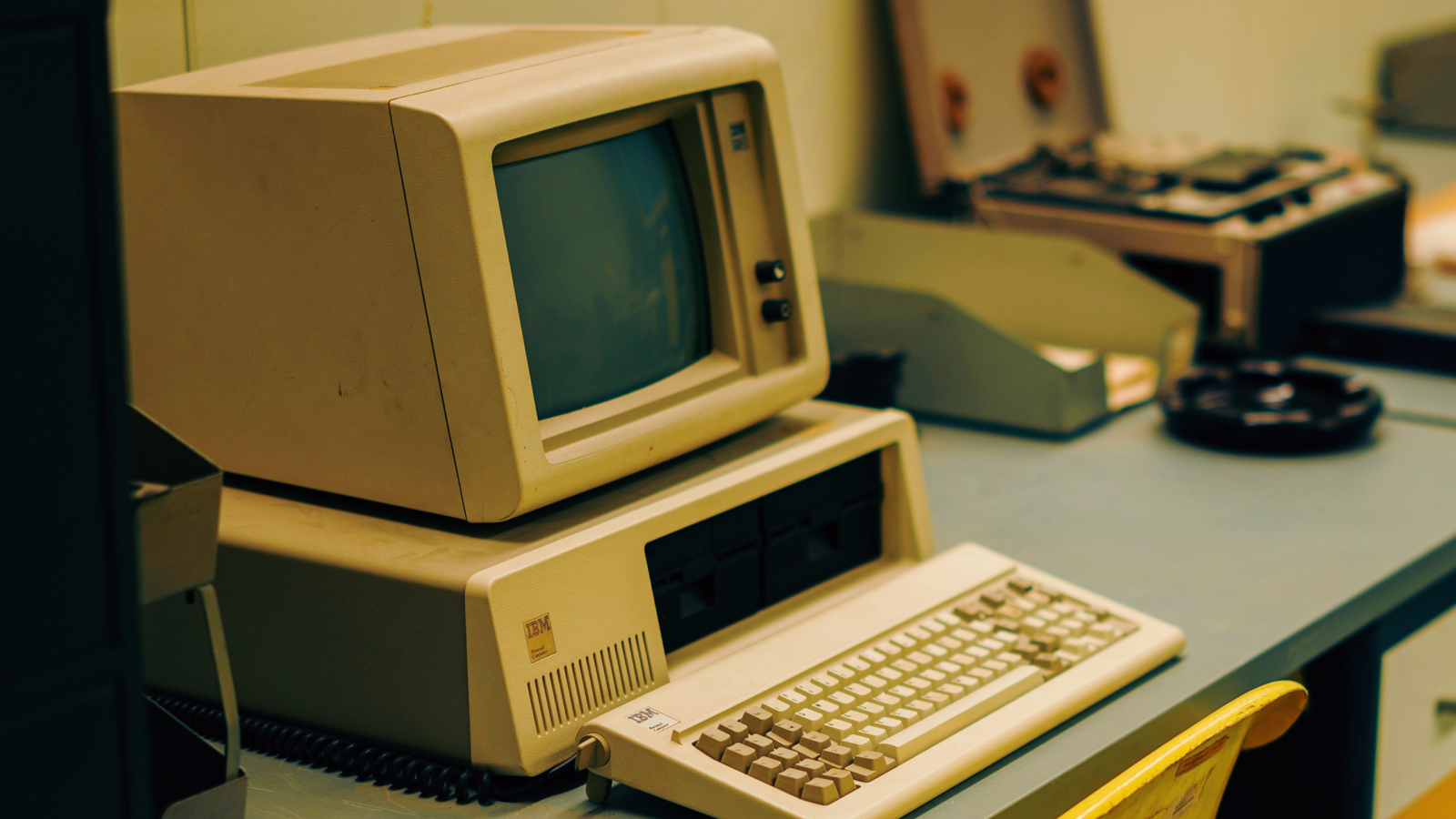
Increasingly sophisticated attacks on the building blocks of 21st-century life — from oil pipelines to power plants and telecommunications — have prompted growing government pressure for industries to strengthen their defenses.
Cyberattacks that led to the shutdown of Seattle’s port and airport and disrupted New York’s emergency services, for example, prompted the NSA and CISA to urge critical infrastructure providers to make improvements as long ago as 2020.
While the response has been slow and businesses are wary of the cost, integrating operational technology that controls physical equipment with information technology that focuses on data management and digital services can help.
Not only does it increase service reliability and avoid disruption, it can unlock opportunities for innovation that boosts revenue, experts told CIO Upside.
“By integrating (Internet-of-Things) and OT systems, you gain visibility into processes that were previously opaque,” Sonu Shankar, chief product officer at industrial security provider Phosphorus, told CIO Upside. Well-managed systems are a “launchpad for innovation,” said Shankar, allowing enterprises to make use of raw operational data.
“This doesn’t just facilitate operational efficiencies — it would potentially generate new revenue streams born from integrated visibility,” Shankar added.
So what counts as operational technology? Any legacy machine, hardware, or device whose operation is essential to a company’s service delivery. That includes anything from factory floor machines to production lines, distribution warehouses, and even smart office printers.
Though updating tech stacks may seem like a daunting task, especially for critical service providers and other industries that rely on legacy hardware, OT-IT management isn’t about breaking the bank. Simple technologies like network segmentation, zero trust security architecture and OT-IT cloud platforms are widely available and cost-effective:
- Network segmentation, the splitting of a main network into many sub-networks, can prevent non-authorized users from accessing critical resources and machines.
- Zero trust architectures, meanwhile, force users to continuously verify themselves to maintain access. This secures digital IT and minimizes human errors and misconfigurations.
- And cloud platforms often provide necessary, centralized visibility that can prevent attacks and offer insight into what may or may not be working within your OT stack. This includes historical detailed reporting, automated maintenance and AI-powered analysis and threat detection.
Fused OT-IT environments lay the groundwork for faster product development and better service delivery, said James McQuiggan, security awareness advocate at KnowBe4. Real-time operational data can feed directly into things like research, development and analytics, he said.
“When OT and IT systems can communicate effectively and securely across multiple platforms and teams, the development cycle is more efficient and potentially brings products or services to market faster,” he said. “For CIOs, they are no longer just supporting the business, but shaping what it will become.”
Microsoft Patents Automate Data Clean-up
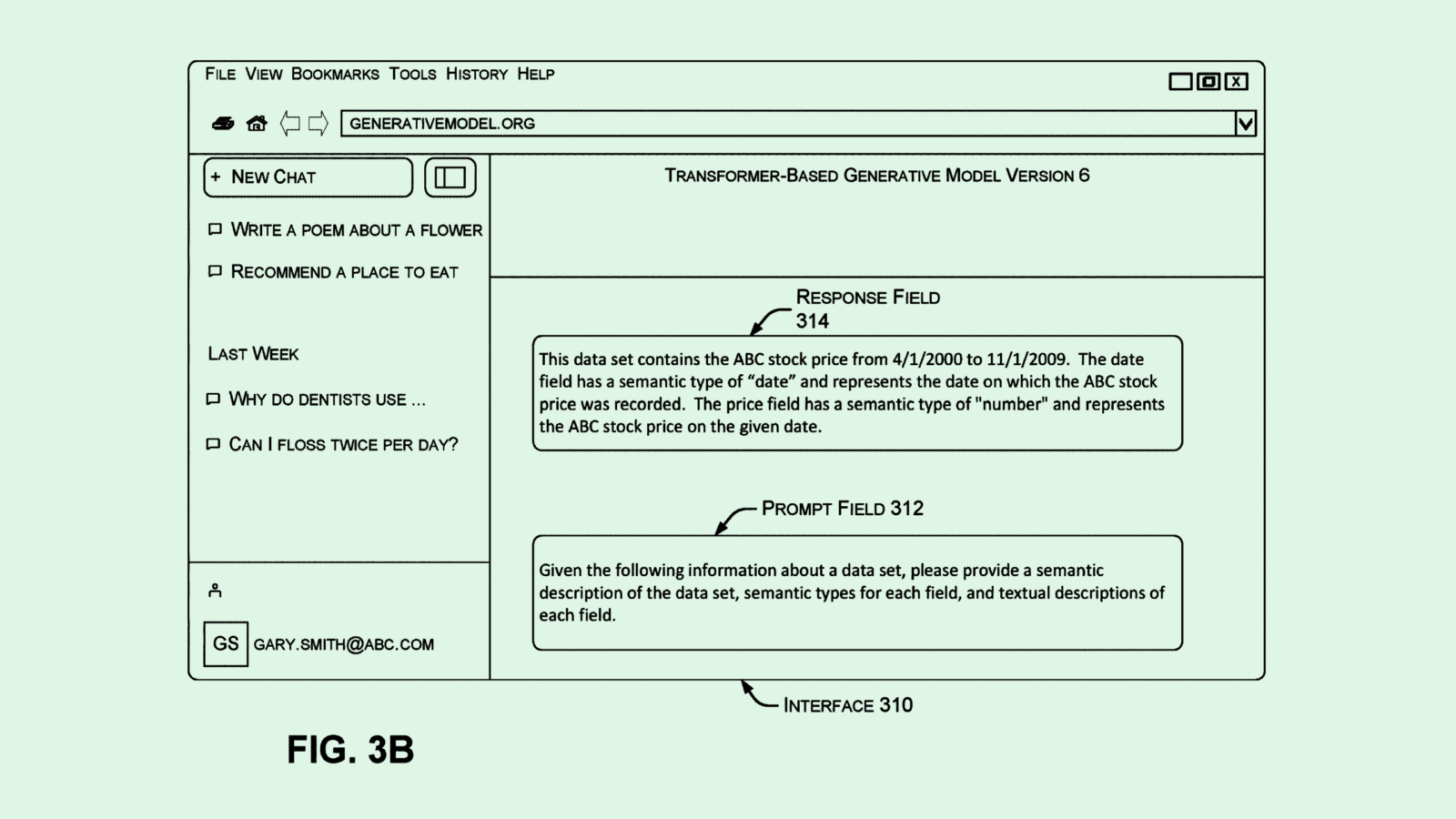
In its quest for AI domination, Microsoft wants to make sure it’s feeding its models well: The company filed two patent applications for tech that uses generative AI to suss out bad data.
First, the company is seeking to patent a system for “data health evaluation” using generative language models that weed out and automatically fixes errors such as missing information or outliers in a dataset without a human needing to audit it.
The system uses an automated agent to prompt the model to create a data evaluation plan, or a list of tests to run on the dataset based on the data itself. Running these checks allows the generative model to find data health issues.
Additionally, the company wants to patent a system that relies on generative models to “improve bad quality and subjective data.” This tech aims to help make sense of subjective data, such as opinions or preferences, that tend to be difficult to label consistently. Instead of a human needing to manually label this kind of data, a generative model essentially interprets that data into something more reliable, which is then used to train smaller and more lightweight models.
“Incorporating subjective data, including bad-quality data, into machine learning models is problematic due to the data’s subjectivity and the challenges in converting it into a usable format,” Microsoft said in its filing.
It’s not the first time data health has come up in patent applications, though data integrity is often tackled from a security perspective, such as Google’s patents to anonymize datasets or IBM’s and Intel’s patents for data minimization techniques.
AI models are only as good as the data they’re built on. With the sheer amount of data it takes to build AI, patents like these seek to automate data integrity and cleanup, a tedious process that can take up a large amount of time for data scientists.
Microsoft could have plenty of uses for tech like this, especially as the company aims to stand on its own two feet in the AI race: Microsoft AI CEO Mustafa Suleyman told CNBC that it’s “mission-critical that long-term, we are able to do AI self-sufficiently.” The quicker it can come up with clean, usable datasets to feed to its AI models, the faster it can stay ahead of competitors.
Extra Upside
- Meta Whistleblower: Sarah Wynn-Williams, Facebook’s former head of Global Public Policy, accused the company of colluding with the Chinese Community Party before the U.S. Senate.
- AI Abroad: The European Commission revealed its “AI Continent Action Plan,” aiming to accelerate AI innovation and development in the region.
- Shopping Spree: PC shipments jumped more than 9% in the first quarter as companies geared up for upcoming tariffs.
CIO Upside is written by Nat Rubio-Licht. You can find them on X @natrubio__.
CIO Upside is a publication of The Daily Upside. For any questions or comments, feel free to contact us at team@cio.thedailyupside.com.