Neuromorphic Computing May Make AI Data Centers Less Wasteful
The technology is garnering interest for its energy-saving benefits.
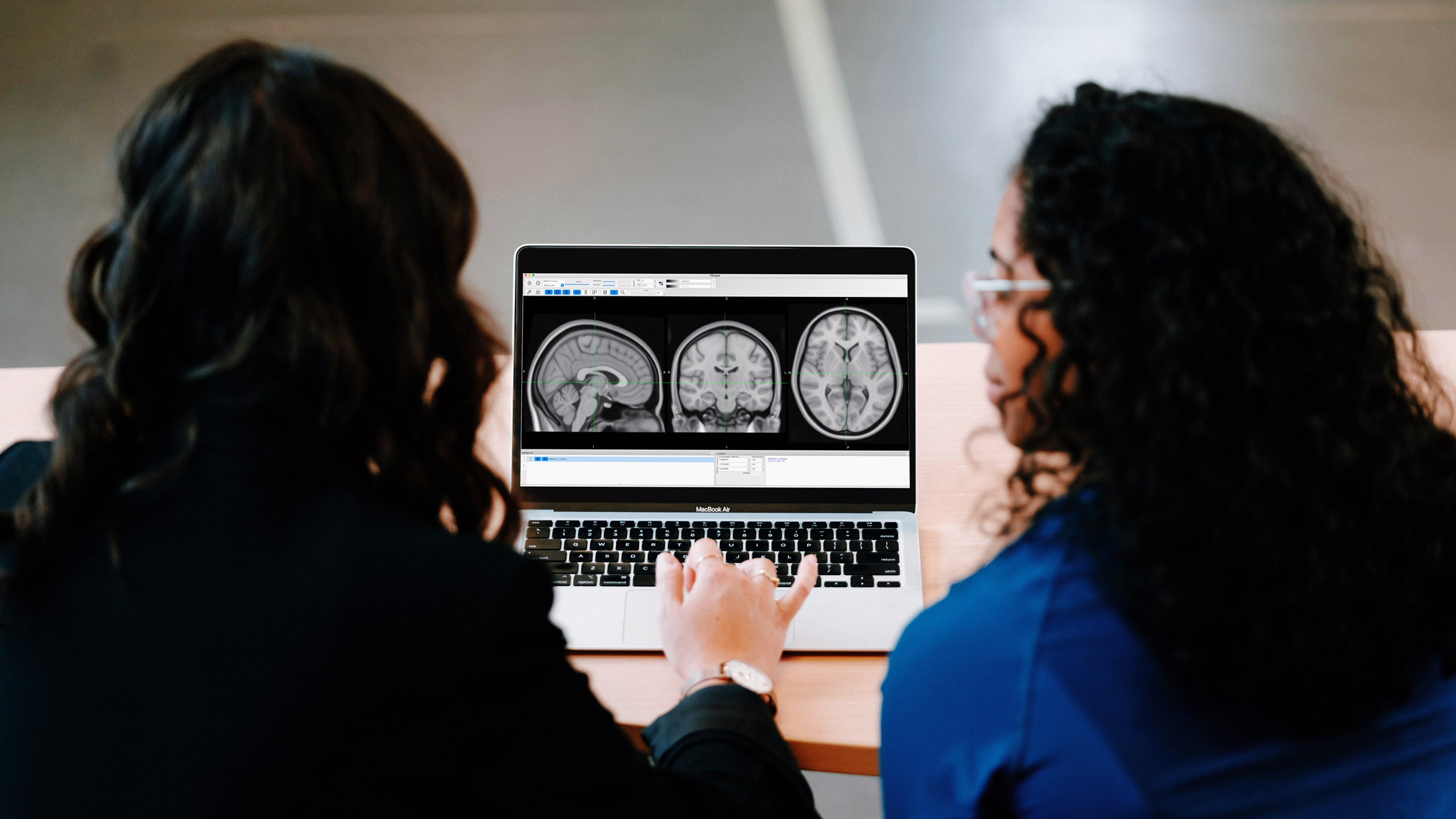
Sign up to get cutting-edge insights and deep dives into innovation and technology trends impacting CIOs and IT leaders.
With AI driving up data center costs and energy usage, tech firms are increasingly interested in alternatives, including one that mimics the human brain: Neuromorphic computing.
Neuromorphic computing processes data in the same location in which it’s stored, allowing for real-time decision making and vastly reducing energy consumption in operations. The tech has the potential to help solve the power problem that continues to threaten the rapid growth of AI, said Sabarna Choudhury, staff engineer at Qualcomm.
Unlike conventional computing systems, neuromorphic computing leverages new hardware such as memristors – a combination of the terms memory and resistor – hardware components that handle processing and storing of data in the same location. That eliminates the energy-consuming processes of transferring data from one place to another.
“By offloading processing tasks to edge devices, neuromorphic tech can reduce data center workloads by up to 90%, enhancing energy efficiency and sustainability while aligning with carbon-neutral goals,” Choudhury said. This approach supports scalable AI without proportional energy increases.
It’s not just data centers that can reap the benefits. Any industry interested in AI stands to gain from the tech, Choudhury said, including aerospace, defense, manufacturing, healthcare, and automotive. Immediate use cases for neuromorphic computing are wide-ranging:
- In healthcare, neuromorphic computing is already being used to support real-time diagnosis and adaptive prosthetics. In the automotive field, neuromorphic vision sensors can provide improved safety.
- In industrial contexts, this tech can enable “real-time quality control and predictive maintenance in robotics, reducing downtime and energy costs,” Choudhury said.
- In military applications, such as DARPA’s SyNAPSE, these systems can be used in creating autonomous, low-power drones and surveillance tech in remote areas.
Neuromorphic computing is also catching the eye of major tech firms: The ecosystem of vendors includes the likes of IBM, NVIDIA, and Intel.
Intel’s Loihi chip, for example, can reduce energy usage by 1,000 times and enable real-time learning for AI deployment, Choudhury said. IBM’s TrueNorth chip also offers neuromorphic, energy-efficient architecture that processes information in real-time with minimal power consumption.
But challenges exist: New algorithms to integrate neuromorphic systems with AI or quantum systems need to be created. And beyond algorithmic integration, the success of neuromorphic computing depends on developing its full stack, from research and innovation in materials to new hardware architectures, circuit designs, and software and applications.
Existing gaps in the supply chain, however, open opportunities for new and established companies that want to innovate in a human brain-inspired tech that could pave the way to a more advanced, efficient, and sustainable AI future, said Choudhury.
“Neuromorphic computing is going to be a necessary step towards advancing AI,” Choudhury said.