Businesses Are Scrapping AI Projects. Here’s How to Find What Sticks
Setting baselines and goals could help companies be more stringent about which AI projects they pick.
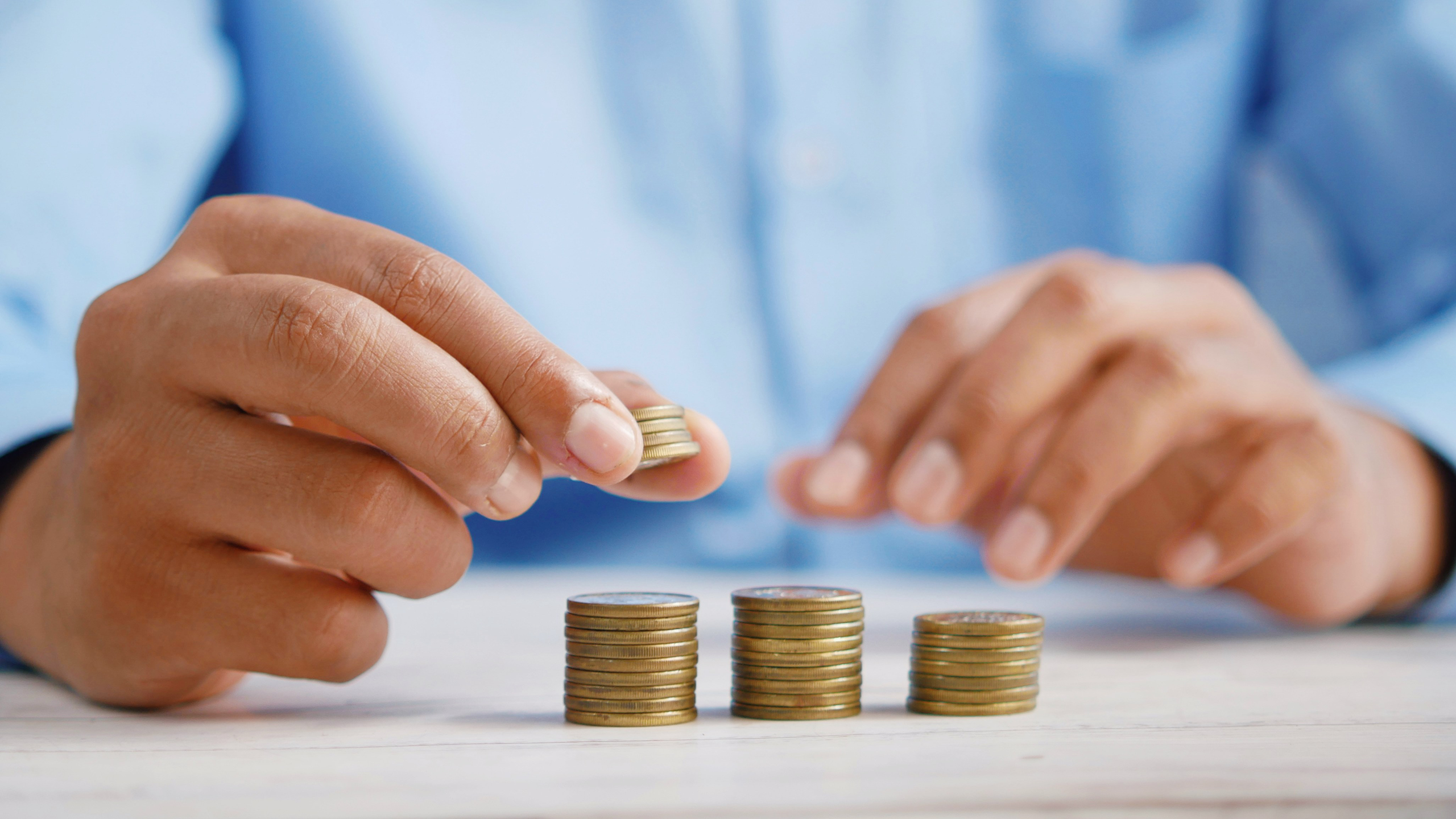
Sign up to get cutting-edge insights and deep dives into innovation and technology trends impacting CIOs and IT leaders.
Enterprises want to take advantage of AI, but figuring out what applications stick is taking a lot of trial and error.
That lack of clarity is illustrated in the accelerating rate at which businesses are dropping AI projects: According to S&P Global Market Intelligence, the number of companies that scrapped AI initiatives grew from 17% in 2023 to 42% in 2024, with the average enterprise cutting 46% of AI proofs-of-concept.
There are a number of reasons that these projects don’t make it out of the “infancy phase,” said Bob Rogers, chief product and technology officer of Oii.ai and co-founder of BeeKeeper AI. For one, a lot of companies are in a discovery phase with AI, in which they’re “throwing spaghetti at the wall and seeing what sticks,” he said.
- Many find that scaling projects outside of small environments or datasets can be quite tricky, said Rogers. “They hit some fairly serious snags with the scruffiness of real life data.”
- Factors like cost, privacy and security issues similarly put pressure on CIOs to be stringent about which AI projects they scale and which ones they scrap, he said.
- Plus, hiring AI teams to actually build and manage these projects is a challenge in and of itself, said Rogers.
That leads many to the “build or buy” equation, said Rogers. While building an internal model that works only on your enterprise’s data can make it more niche, the difficulty of scaling may lead these firms “more in the buy direction,” he said, choosing models or AI integrations offered by outside sources. “The maturity of offerings coming from outside is going to be better,” said Rogers.
Internal projects need to make “that leap of making security, reliability, integration with other tools industrial-strength,” Rogers said.
So how can enterprises have better AI sticktoitiveness? One way is to set benchmarks from the start of a project, said Rogers. If you don’t know what exact metrics your AI project can improve, then figuring out whether or not a project will see returns in the long run is all the more difficult.
“Benchmarking is a critical component,” said Rogers. “If you don’t have as part of your plan a benchmark of what it is that you’re really improving … if you hit some tough sledding, it’s really, really hard to keep the momentum.”
Having the foresight to account for security problems and the “weirdnesses” of real-life data can also help enterprises get their AI projects out of the sandbox, Rogers said. “None of the AI projects that are hypothetically thrown away are doomed to failure for sure,” he said.
“Quite often there’s actually an opportunity to move forward,” said Rogers. “But the wherewithal of an organization to see through that noise and get to the next level is very challenging.”