Google Patent Keeps Your Data Under Wraps
Plenty of other tech giants have sought to solve the ever-present AI data security problem.
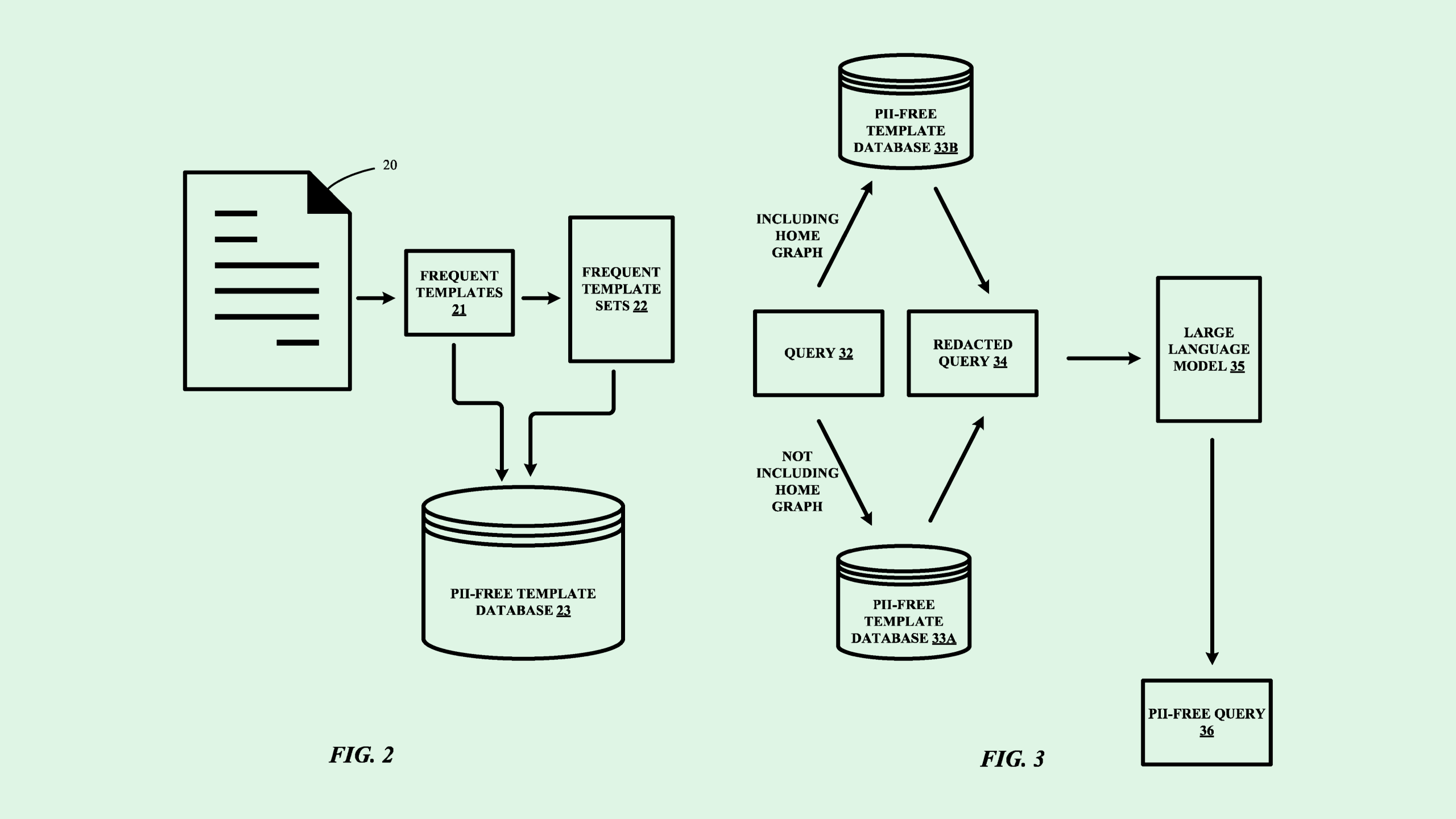
Sign up to get cutting-edge insights and deep dives into innovation and technology trends impacting CIOs and IT leaders.
Large language models still have larger data security issues.
Google may have a solution: The company filed a patent application for “robust pseudonymization that retains data diversity.” To put it simply, this tech automatically redacts personally-identifiable information from queries sent into an AI model, keeping sensitive information from reaching the model in the first place.
“If a model is trained using training data that includes (personally identifiable information), it may be possible for a malicious user to retrieve that PII by asking specific questions of the automated assistant,” Google said in the filing.
This system relies on a bank of frequently used phrases or words in what Google calls a “PII-free template database.” Then, when a user submits a query that includes personally identifiable information, such as full names, emails or addresses, a generative model uses that bank to recreate the query, checking the original input against the PII-free database. The new query, which has placeholders where any personal data would be, is then fed to the model and used for training.
Plenty of other tech giants have sought to solve the ever-present AI data security problem: Microsoft filed a patent to prevent so-called AI “jailbreaks,” and Intel sought to patent a way to verify that a model hasn’t been tampered with post-deployment. Google, meanwhile, has sought to patent a way to entirely anonymize large-scale datasets before they even reach models.
As these patents highlight, AI still faces massive cybersecurity problems. Adoption, however, often moves faster than the implementation of security guardrails. The problem may get worse as AI agents come onto the scene: Giving these models more autonomy can cause data security domino effects that have a higher risk of going unnoticed.
The tradeoff of relying on anonymous or synthetic data, however, is often usability and model accuracy. Finding the right balance means approaching security from multiple angles – thinking not just about the data and inputs themselves, but the environment in which your model is deployed.