Need Help Finding Security Blind Spots? Look Beyond Your ‘Red Team’
You can’t fix what you can’t find.
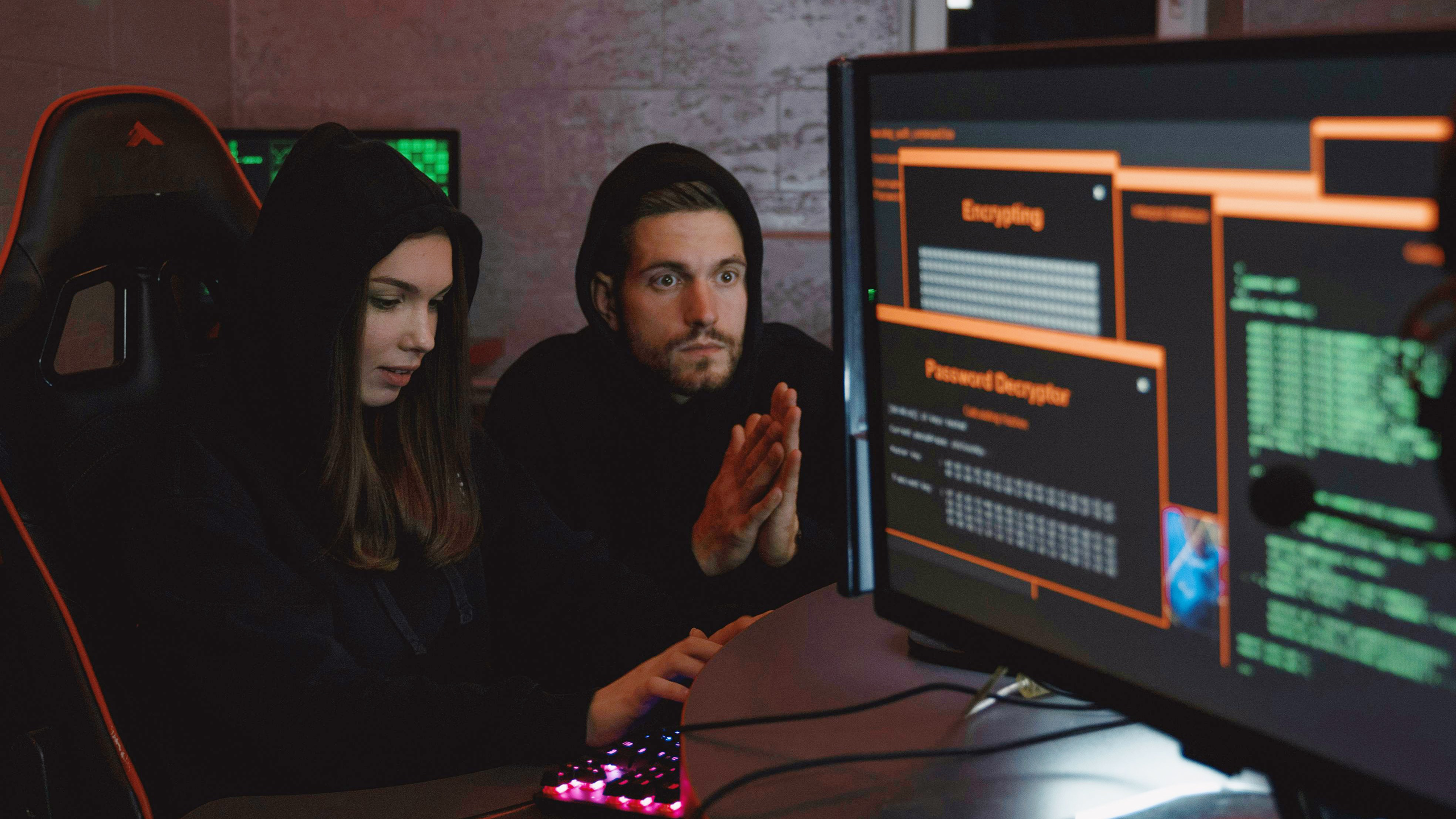
Sign up to get cutting-edge insights and deep dives into innovation and technology trends impacting CIOs and IT leaders.
When it comes to security, you need to know what you don’t know.
In order to properly manage security vulnerabilities, enterprises need to take inventory of where, exactly, their pitfalls exist – especially as AI creates a broader area of risk. But relying only on internal “red teams,” or groups dedicated to simulating attacks on an AI model, may not be enough to identify all the hazards, said Dane Sherrets, staff innovation architect at HackerOne.
As organizations attempt to move quickly on AI adoption and development, the increasingly complex job of security can often fall on small and strapped cybersecurity teams, where flaws can fall through the cracks. These flaws can have massive domino effects. “Having more safety and security leads to more trust, which can lead to more adoption, which leads to more innovation,” said Sherrets.
Instead of relying only on internal teams to poke and prod your AI models, being “open and willing to engage with the researcher community” could reveal more about your organization’s security stumbling blocks than a single red team is capable of, said Sherrets.
- Realistically, limits on the size of an internal team, the resources of an enterprise and the diversity of members’ backgrounds can all be hindrances to finding every pitfall possible, he said.
- “To really poke and prod these models, you want to have diversity of backgrounds – and of people,” said Sherrets. “There is sort of a magic that happens when you invite people to bring in new techniques.”
While enterprises should have internal teams dedicated to identifying flaws in AI systems, that shouldn’t be the “end-all, be-all,” said Sherrets. A recent paper by Sherrets and a group of AI researchers across 24 organizations found that current flaw-reporting systems for models have major gaps, with problems often going unreported due to a lack of proper infrastructure.
Flaws in large-scale, general-purpose AI systems can pose massive risks to the consumers, developers and enterprises that use them. But building proper channels, such as standardized reporting systems, bounties and legal protections, can “incentivize researchers such as myself to spend the hours and weekends diving in deep,” he said.
“All risk management begins with an inventory,” said Sherrets. “This will allow for a bigger and better inventory.”
So where should enterprises start? If you’re building AI, the first step is making sure you have internal resources in place to accept feedback on the flaws of your systems – and personnel in place to actually do something about them, said Sherrets.
“People are going to find stuff,” Sherrets added. “So eat your vegetables first. Make sure you have teams that can own remediation and a process for acting on (flaws). Make sure you’re operationally ready.”